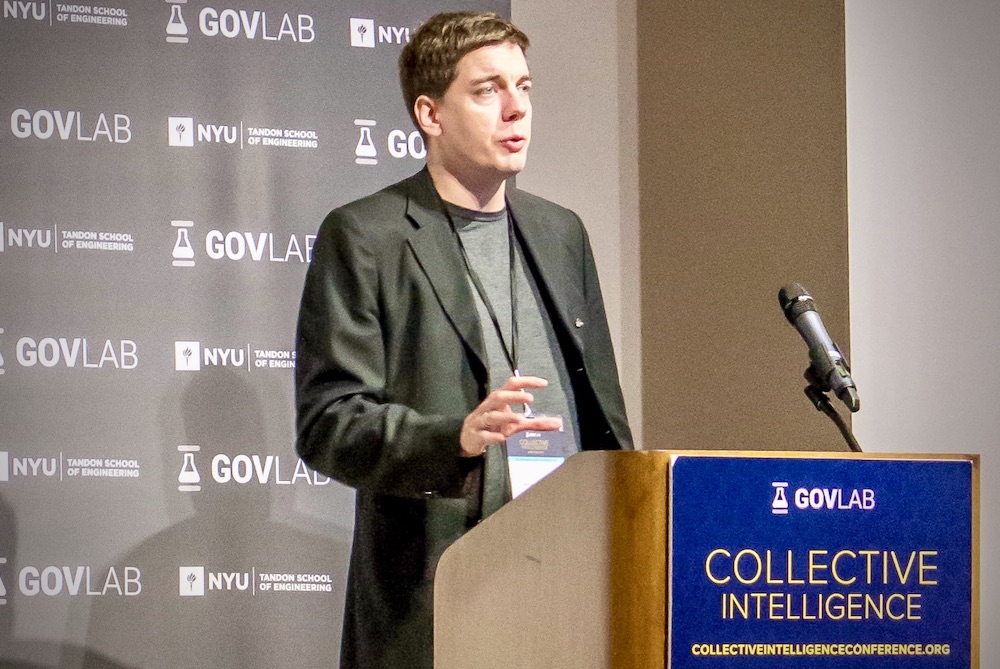
Spearheading the CSSLab’s work on high-throughput virtual lab experiments on group dynamics, Mark Whiting is helping to define the paradigm of large-scale, data-driven social science research. In this researcher spotlight, he outlines his research trajectory and thoughts on the future of CSS.
Q: Tell me a bit about yourself and your research background.
A: My research background has covered a lot of different areas with a common thread. My parents are a physicist and an archeologist, and I think this led me to pursue precise models of the world and ways to understand it carefully and systematically. But, at the same time, I’m interested in applying those approaches to the kinds of things that are really hard to measure and understand: people, especially people of whom you can’t ask things directly.
I initially approached this vision in my undergrad and masters in industrial design. I enjoyed both technical and humanities fields, and I thought that a good way to find a junction between those is to build things for the world. Design seemed like a great way to do that.
After working in design for a few years, though, I found that it leans away from seeking truth about the world and more toward applying rules of thumb. So I pursued my Ph.D. because I thought there could be an interesting direction that was a bit more scientific, where we might understand with more nuance what people are really doing and really thinking about—and perhaps make better design decisions.
I did my Ph.D. in mechanical engineering with a group that specialized in automated/engineering design. Basically, people in this field apply mathematical techniques and modeling approaches to questions about how people decide things, particularly in the context of design decisions. My Ph.D. work was about how we can automate some of those decisions and whether or not we can apply that automation to other contexts.
Through this process, I realized that to be successful at this goal would also require a means of understanding humans and how they interact. As in, I’d need to bounce back and forth between more human or more technical approaches. The field in which I have settled—broadly, computational social science, and more specifically, HCI [human-computer interaction] applied to social scientific research questions—is really supportive of this balance, and full of people with a similar alignment.
One thing that has been overwhelmingly important to me over the past few years is how individuals turn into societies. Any one member of a society has all these individual attributes and behavioral aspects, but they also have groups that they’re in, and those groups all have their own separate behaviors and ways of making decisions. So it turns into a very interesting multi-scale problem where we might design a new platform—like Twitter—and it might tell us something about what’s happening to people, but it’s often very hard for us to pinpoint where these different scales are influencing what we’re seeing.
A lot of my projects build on that undercurrent in some way. For example, at Stanford, we tried to understand if teams fall apart due to who’s on the team or how the team is orchestrated—a classically intertwined pair of causes in social psychology, person and situation. Traditionally, there has not been a good experimental infrastructure to disaggregate these two in this context, so we built an experimental collaboration platform in which group member identities were strategically masked across repeated interactions; people think they are on a team with new collaborators when they are actually there with the same ones.
In effect, this lets us reset collective memory, so it is possible to see if group outcomes within a particular group collaboration are a consequence of who’s on the team or how the group is orchestrated. There was no experimental infrastructure in place that let researchers examine these two notions separately, so we built it ourselves and have gone on to use it in a number of other studies including to predict a team’s success, to intervene when teams are having a hard time, and to study if teams or individuals have more consistency in moral decisions.
Q: How did you become involved with the CSSLab?
A: I was finishing up my time at Stanford and was interested in doing more research in this space. Duncan [Watts] had done some work years ago that served as a partial inspiration for some of our techniques in the teams platform I built at Stanford, so he was an obvious person to reach out to. Duncan and I talked, and we were very excited about a couple of projects that ended up being the two main projects that I now work on at the Lab: understanding common sense and high-throughput teams.
Q: You’ve mentioned a few things that you’re working on now. Tell me more about those projects.
A: I work on lots of different things with lots of different moving parts. At a high level, much of my work is around teams and team dynamics, and how we’re studying team dynamics using the high-throughput teams project. That is, of course, a very long and ongoing project, and much of what I’ve been doing has been in orchestration, platform building, infrastructure, and research design. One of the main pillars of our work right now is building this massive research panel and a semi-automated research infrastructure to run it. We’re also doing a lot of work on mapping the research domain, which has been a huge undertaking for several years.
My work on common sense is this other project where we’re trying to understand a broad question: how common is “common sense,” actually? There is this common intuition that it’s everywhere and that everyone has some of it. But we also use common sense as a distinguishing factor between people who don’t think similarly, where we can tell someone who has differing views that they don’t have common sense. In that case, which happens a lot in rhetorical situations like political discourse, what you’re really implying is that common sense is your opinion as opposed to what you think everyone else thinks. So what is common sense really? In both these projects there’s this huge challenge of trying to pin down and understand concepts that are usually used by science and society in a problematically vague way.
COVID is another area we’re working on. Most of my work there has been around dashboards and building tools for the City of Philadelphia, which they can use to try and think about vaccination or shutdown strategies. The nature of the situation is changing so rapidly that it’s hard to build robust tools that can adapt to the city’s needs, but we’ve delivered a few that are being tested out.
Q: In your current work, what are the biggest challenges you’ve run into, or the most rewarding parts of it so far?
A: The biggest challenge might have been that I started just before COVID. I don’t think I would change anything, given the circumstances, but I definitely thought that I would interact with Duncan and the team a lot more in person, and that a lot of the experience overall would be really different. The main challenge with COVID has not necessarily been that we can’t do our work; it’s more that it’s harder to get interpersonal context. But I think we’ve worked our way around that, and we now have quite a large and powerful group doing lots of cool things.
Another big challenge is that these kinds of massive research projects often sound straightforward. When we talk about them, we can abstract away a lot of the nuance of actually doing them. We all really thought it would be much faster and simpler, and I think a big part of that difficulty is that it’s so easy to overlook the real-world hurdles of building these systems. But we’re increasingly close to being finished, and are constantly battling with reality.
One of the exciting things about doing that is that every time you solve a problem that was very hard for you to solve, it’s typically valuable to the world. Of course we don’t have the time to write up every solved problem as a paper, but certainly many of them can become papers that are really useful to others at some point. We find it exciting to think about how each of these instances of tackling a huge problem can be beneficial to the institution of science going forward.
Q: What’s in store for your projects?
A: My two main projects—high-throughput teams and common sense—are large and exciting ways to think about future research that I want to do. My hope is that we can continue to work in the spaces that we’re defining around them. As an example of that, our work on common sense is all about how you measure society-level concepts that have cross-sectional value at different scales. You could have individual-level common sense, community-level common sense, or society-level common sense. And measuring something like a society-level construct—which also has this intriguing property of how individuals play into it—has traditionally been a little tricky, especially with concepts that are almost philosophical in nature. So that project, to me, embodies trying to measure philosophical attitudes as opposed to trying to mandate them, and we’re learning how to model these hard-to-measure concepts at a very large scale.
And similarly, with the teams project, it’s a very big first step, but it’s a first step in a way of thinking about lots of elements and kinds of research that I think could be really exciting moving forward. For example, we’re wondering if you can design experiments that have some of the properties of this massive high-throughput-style science in real-world and non-experimental contexts. Another direction is that we can consider what other kinds of questions we can answer using these high-throughput experimental techniques.
So if we’re asking where I see all this work in, say, ten years’ time, I think all of it is stuff that I’m going to be excited about for a long time, and there are many alternative directions for us to move in. I think even beyond these individual projects, though, working with Duncan and with this group has been really exciting because Duncan has made huge projects for basically his whole career. I think there are many parts to his method that are probably not easily intuited by other people trying to do this kind of research, so I feel that I’ve learned a lot about that process and how to move forward—in particular, things like being extremely, extremely careful about what you assume from your data, doing a ton of double-checking to ensure that every aspect of what you’re seeing is really what you’re seeing, and being clear about your definitions to a level that I think is actually very challenging and rare in research today.
Learn more about Mark’s current research by visiting our Group Dynamics and Common Sense project pages.
AUTHORS
EMMA ARSEKIN
—
Communications Specialist