“Half the Truth is often a great Lie.”
– Benjamin Franklin
PennMAP
Penn Media Accountability Project
PennMAP is an interdisciplinary, nonpartisan research project of the Computational Social Science Lab at the University of Pennsylvania dedicated to enhancing media transparency and accountability at the scale of the entire information ecosystem.
Touchstone Publications
MISUNDERSTANDING THE HARMS OF ONLINE MISINFORMATION
QUANTIFYING THE IMPACT OF MISINFORMATION AND VACCINE SKEPTICAL-CONTENT ON FACEBOOK
CAUSALLY ESTIMATING THE EFFECT OF YOUTUBE’S RECOMMENDER SYSTEM USING COUNTERFACTUAL BOTS
Data Dashboard:
Media Bias Detector
To measure and expose bias in the mainstream media, PennMAP is proud to launch the Media Bias Detector, which tracks and classifies the top stories published by a collection of prominent publishers spanning the political spectrum in close to real time.
DASHBOARDS
Mission
Misinformation in media is believed to have harmful effects on public opinion, political polarization, and ultimately democratic decision making. And yet, much remains unknown regarding the prevalence of misinformation and its effects on society.
To address this problem, PennMAP is building technology to detect patterns of bias and misinformation in media from across the political spectrum and spanning television, radio, social media, and the broader web. We will also track consumption of information via television, desktop computers, and mobile devices, as well as its effects on individual and collective beliefs and understanding.
In collaboration with our data partners, we are also building a scalable data infrastructure to ingest, process, and analyze tens of terabytes of television, radio, and web content, as well as representative panels of roughly 100,000 media consumers over several years. While our initial focus is on the U.S., our hope is to scale this infrastructure to eventually cover other countries and languages other than English.
Commitment to Use-Inspired Research
We will share our insights through publications and interactive data visualizations, and will work with various stakeholders—including journalists, policy makers, and industry partners—to implement solutions. Aside from powering our own research, our infrastructure will support other research teams, thereby accelerating the pace of knowledge accumulation and enhancing its reliability.
In the News
Duncan Watts and CSSLab’s New Media Bias Detector
The 2024 U.S. presidential debates kicked off June 27, with President Joe Biden and former President Donald Trump sharing the stage for the first time in four years. Duncan Watts, a computational social scientist from the University of Pennsylvania, considers this an ideal moment to test a tool his lab has been developing during the last six months: the Media Bias Detector.
“The debates offer a real-time, high-stakes environment to observe and analyze how media outlets present and potentially skew the same event,” says Watts, a Penn Integrates Knowledge Professor with appointments in the Annenberg School for Communication, School of Engineering and Applied Science, and Wharton School. “We wanted to equip regular people with a powerful, useful resource to better understand how major events, like this election, are being reported on.”
What Public Discourse Gets Wrong About Misinformation Online
Researchers at the Computational Social Science Lab (CSSLab) at the University of Pennsylvania, led by Stevens University Professor Duncan Watts, study Americans’ news consumption. In a new article in Nature, Watts, along with David Rothschild of Microsoft Research (Wharton Ph.D. ‘11 and PI in the CSSLab), Ceren Budak of the University of Michigan, Brendan Nyhan of Dartmouth College, and Annenberg alumnus Emily Thorson (Ph.D. ’13) of Syracuse University, review years of behavioral science research on exposure to false and radical content online and find that exposure to harmful and false information on social media is minimal to all but the most extreme people, despite a media narrative that claims the opposite.
Mapping Media Bias: How AI Powers the Computational Social Science Lab’s Media Bias Detector
Every day, American news outlets collectively publish thousands of articles. In 2016, according to The Atlantic, The Washington Post published 500 pieces of content per day; The New York Times and The Wall Street Journal more than 200. “We’re all consumers of the media,” says Duncan Watts, Stevens University Professor in Computer and Information Science. “We’re all influenced by what we consume there, and by what we do not consume there.”
In the News
Duncan Watts and CSSLab’s New Media Bias Detector
What Public Discourse Gets Wrong About Misinformation Online
Mapping Media Bias: How AI Powers the Computational Social Science Lab’s Media Bias Detector
Mapping How People Get Their (Political) News
The CSSLab Launches News Consumption Dashboard
Misleading COVID-19 headlines from mainstream sources did more harm on Facebook than fake news
People
Duncan Watts
Stevens University Professor
Penn Integrates Knowledge Professor
Homa Hosseinmardi
Associate Research Scientist
Amir Ghasemian
Affiliate Research Scientist
Yale University
David Rothschild
Affiliate Research Scientist
Microsoft Research
Coen Needell
Pre-Doctoral Researcher
Timothy Dorr
Ph.D. in Communications
Upasana Dutta
Ph.D. in Computer and Information Science
Samar Haider
Ph.D. in Computer and Information Science
Baird Howland
Ph.D. in Communications
Sam Wolken
Ph.D. in Communications and Political Science
People
Duncan Watts
Stevens University Professor
Penn Integrates Knowledge Professor
Homa Hosseinmardi
Associate Research Scientist
Amir Ghasemian
Affiliate Research Scientist
Yale University
David Rothschild
Affiliate Research Scientist
Microsoft Research
Coen Needell
Pre-Doctoral Researcher
Timothy Dorr
Ph.D. in Communications
Upasana Dutta
Ph.D. in Computer and Information Science
Samar Haider
Ph.D. in Computer and Information Science
Baird Howland
Ph.D. in Communications
Sam Wolken
Ph.D. in Communications and Political Science
Publications
Misunderstanding the harms of online misinformation Journal Article
In: Nature , vol. 630 , pp. 45-53, 2024.
Quantifying the impact of misinformation and vaccine-skeptical content on Facebook Journal Article
In: Science, vol. 384, iss. 6699, 2024.
Deplatforming did not decrease Parler users’ activity on fringe social media Journal Article
In: PNAS Nexus, vol. 2, iss. 3, 2023.
Quantifying partisan news diets in Web and TV audiences Journal Article
In: Science Advances, vol. 8, iss. 28, 2022.
Reducing opinion polarization: Effects of exposure to similar people with differing political views Journal Article
In: Proceedings of the National Academy of Sciences, vol. 118, no. 52, 2021.
Success stories cause false beliefs about success Journal Article
In: Judgment and Decision Making, vol. 16, no. 6, pp. 1439-1463, 2021, ISSN: 1930-2975.
Comparing Estimates of News Consumption from Survey and Passively Collected Behavioral Data Journal Article
In: Public Opinion Quarterly, 2021.
Examining the consumption of radical content on YouTube Journal Article
In: Proceedings of the National Academy of Sciences, vol. 118, no. 32, 2021.
Research note: Examining potential bias in large-scale censored data Journal Article
In: Harvard Kennedy School (HKS) Misinformation Review, 2021.
Measuring the news and its impact on democracy Journal Article
In: Proceedings of the National Academy of Sciences, vol. 118, no. 15, 2021.
Evaluating the fake news problem at the scale of the information ecosystem Journal Article
In: Science Advances, vol. 6, no. 14, pp. eaay3539, 2020.
Understanding Cyberbullying on Instagram and Ask.fm via Social Role Detection Journal Article
In: Companion Proceedings of The 2019 World Wide Web Conference, pp. 183-188, 2019.
Evaluating overfit and underfit in models of network community structure Journal Article
In: IEEE Transactions on Knowledge and Data Engineering, vol. 32, no. 9, pp. 1722-1735, 2019.
The science of fake news Journal Article
In: Science, vol. 359, no. 6380, pp. 1094-1096, 2018.
Beyond Keywords: Tracking the evolution of conversational clusters in social media Journal Article
In: Sociological Methods & Research, vol. 48, no. 3, pp. 588-607, 2017.
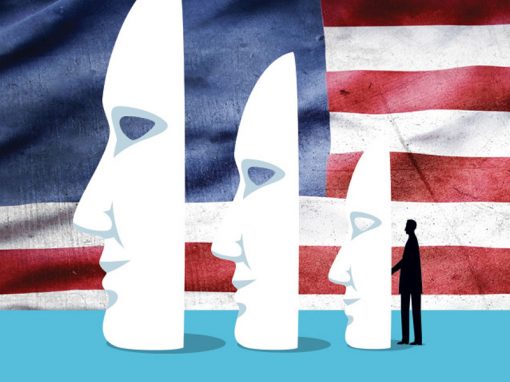
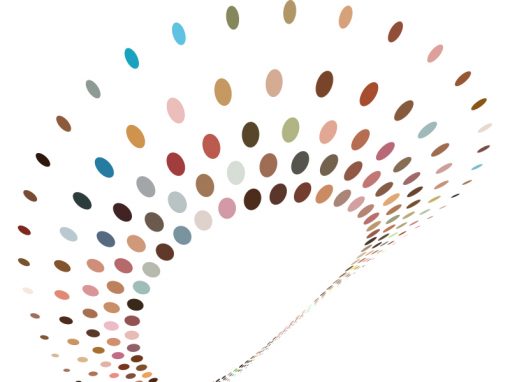
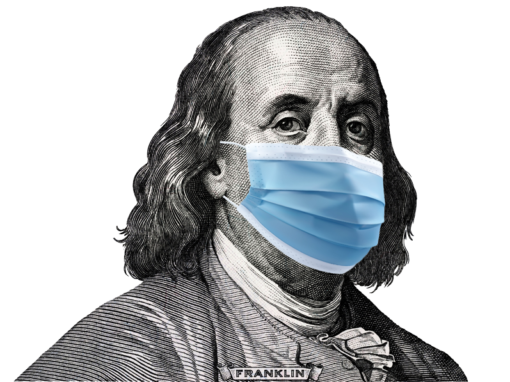
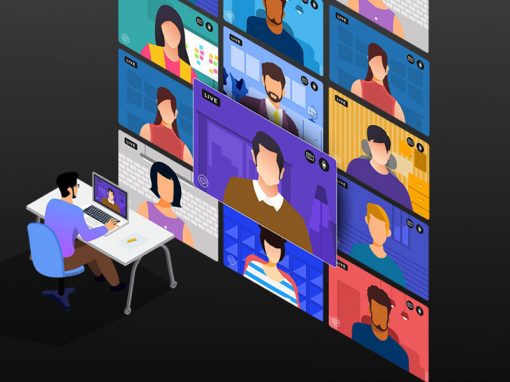
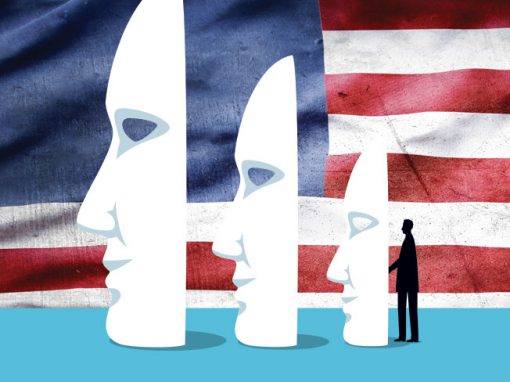