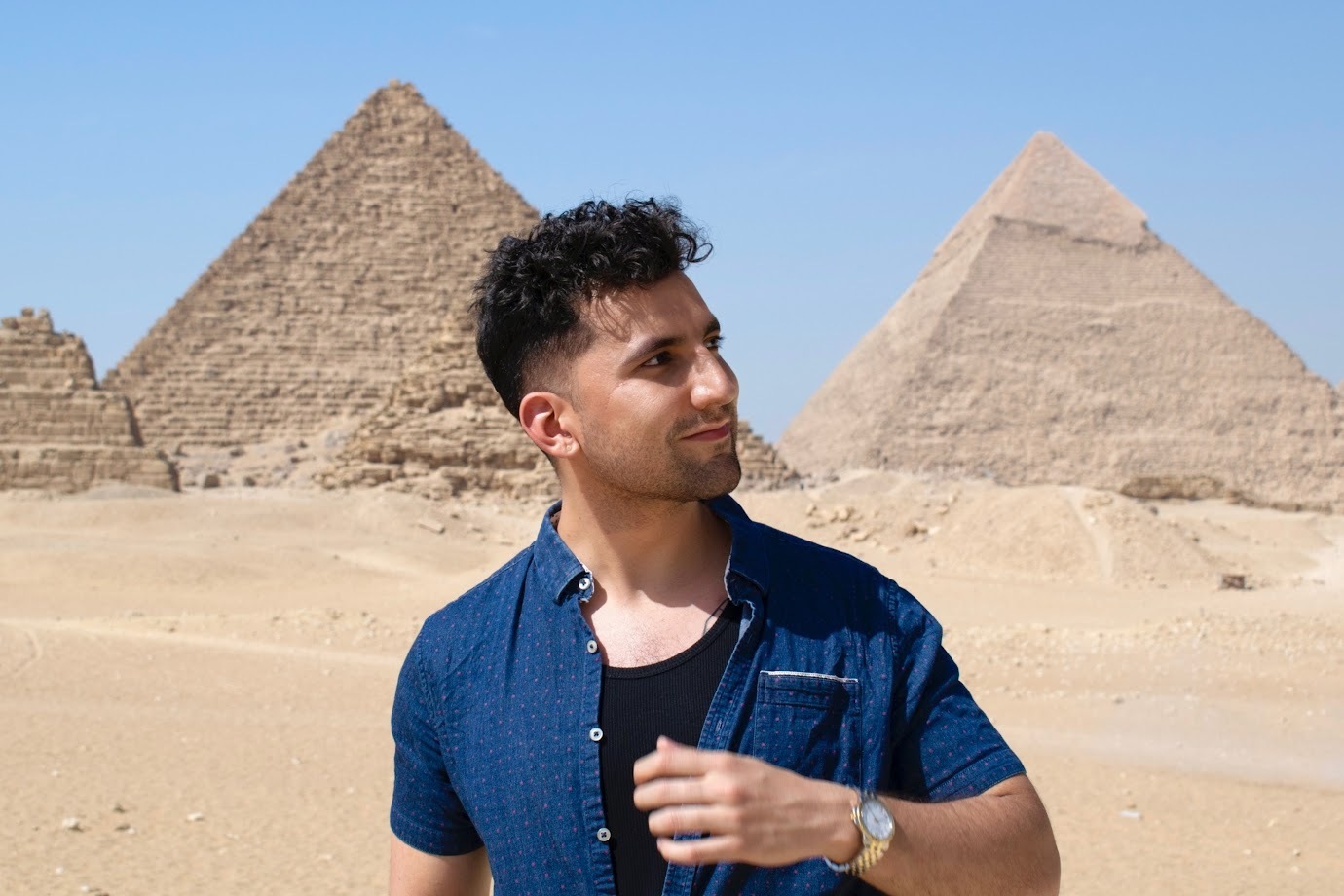
It’s a data scientist’s world, but it’s nothing without a mathematician: Having completed his Ph.D. in applied math, Jorge “Paco” Barreras Cortes will be continuing his work at the CSSLab as a fully fledged post-doctoral researcher.
Having just earned his Ph.D. in applied math in December, Jorge “Paco” Barreras Cortes kicks off 2023 as a fully fledged post-doctoral researcher at the CSSLab. He has driven the Lab’s work on epidemic modeling since 2020, grappling with the types of data, machine learning, and network science quandaries that underpin the toughest challenges in the field. Read on to learn more about his research journey in this month’s Researcher Spotlight.
Q: First, tell me a bit about yourself and your background.
A: I completed my bachelor’s degree in math and economics, along with my master’s in economics, at the Universidad de los Andes in Bogotá, Colombia. I didn’t know whether I wanted to enter academia afterwards, so I worked for a few years to explore the types of work I might be interested in.
My thesis advisor for my master’s launched a consulting company, so I started there and wrote a few papers. Then I worked for the Bogotá lottery, of all things, and with Colombia’s financial intelligence agency, where I first got to experiment with natural language processing. I also cut my teeth on spatial data through a project to find illegal mining operations using satellite imagery. (What we found, more than anything, was that the Colombian census of illegal mining isn’t great — we’d sometimes check their coordinates and they’d land us in the middle of the Pacific, or in another country altogether.)
After these experiences, and motivated by the desire to prove to myself that I could do “real” mathematics, I started an applied math Ph.D. I chose Penn out of a few other top schools because of the flexibility of its program; it gives you a lot of freedom to collaborate with top researchers once you learn your fundamentals.
Q: How did you become involved with the CSSLab?
A: I attended a lecture at NetSci [Network Science Society conference] 2018, where Duncan [Watts] was a keynote speaker. I was impressed by his talk and learned that he was soon moving to Penn, so I thought of approaching him and work with him in some way. My thesis proposal defense was coming up — I knew I wanted to do something related to processes in networks, so I figured I’d ask him to be in my committee.
I approached Duncan with an initial proposal about polarization in information networks that, in retrospect, was a bit naive. It had interesting math ideas, but he quickly asked what my data was going to be, and wasn’t particularly excited about using Twitter data. I had included a snippet at the end of the proposal on epidemics, however. He found that more interesting and, after a bit of a back and forth, I got him interested on a completely new proposal centered on epidemics.
After putting together this new proposal, titled “Data-driven control of epidemics,” COVID started, and Duncan and my co-advisors suddenly became very interested in my topic. What was supposed to be a one hour per year commitment transformed into Duncan calling me into his office the next day, and that turned into us meeting once a week. I wasn’t ever really his advisee, but he stepped into the advisor role really well.
“At the beginning, we were just working with the epidemic models that people were using at the time […] Then, as you might imagine, COVID changed everything.”
Q: Tell me about the COVID project you started working on when you joined the Lab.
A: At the beginning, we were just working with the epidemic models that people were using at the time — essentially gigantic simulators where you plug population info and the properties of the virus and you press “play.” Then, as you might imagine, COVID changed everything. Duncan suddenly got a lot of contact requests from companies who wanted us to use their location data in our work. But these datasets are huge, complex, and full of subtleties; you can’t just plug them into the previous idea we had.
As we were figuring out how to integrate all this new data, Mark [Whiting] made some beautiful dashboards for the City of Philadelphia showing some mobility metrics I had computed. It seemed very cool and useful for epidemic tracking purposes. But in exploring those dashboards, we started to realize that the data had artefacts.
Major ones.
In that we would look at, for example, a pharmacy, and the data showed only two visitors to that pharmacy in a whole month, and they both came from the other side of town. The more we stared at the data, the more of these bizarre anomalies we saw, and we slowly became more and more absorbed in figuring out what was happening beneath the surface.
Q: Was there a particular anomaly in the data that made you stop in your tracks?
A: We focused a lot on one metric, which we visited over and over again, that seemed like an amazing way to measure social distancing: the percentage of people who did not leave home at all on a given day. People would use this metric in their models and in impactful research, and governments would cite papers that showed a positive relationship between social distancing mandates and the number of people staying home. But there were also people who used that metric to argue the opposite, saying that the data wasn’t correlated with COVID caseloads, so the mandates were not necessary or effective.
So people on both sides were using the same metric to argue for very high-stakes claims. And when we actually looked at the data behind it, we found some concerning stuff. In all of 2019 — when there was no pandemic — we found, for example, many neighborhoods where 80% of the residents were not leaving home at all. And it’s really hard to show that that’s wrong, because we don’t have that type of data at a daily granularity from anywhere else to cross-check as “ground truth.” Moreover, this same metric looked very different between two different datasets. So at least one of them was wrong, or it was a signal that both were noisy and biased versions of the truth.
“People on both sides were using the same metric to argue for very high-stakes claims. And when we actually looked at the data behind it, we found some concerning stuff.”
Q: What did you uncover when you examined the data more deeply?
A: After a year and half of analyzing the data, we learned a few things. First, compared with external data sources, the data was indeed anomalous. For example, there are surveys of how many people commute to work every day. That’s not the same as staying home every day, but we can deduce that if someone’s commuting every day, they’re not staying at home. And those numbers did not line up with our data at all.
Second, the data was incredibly sparse. For a given user — the building block of all these sophisticated metrics and high-stakes claims — sometimes we’d only see their activity in the data for 10% of the hours in a week. What do you do with all those huge gaps? Do you exclude people? Do you try to fill in missing information using math? With just a few pinpointed filters to try to get higher quality data, you’re left with only a handful of data points that you can use with any remote accuracy. Whether you use that subset or any other subset along the way impacts your results drastically.
Finally, we realized that behind all the useful metrics we can glean from this data — how much time people spend at home, the distance they travel, the number of locations they visit, the radius of the area they’re from, the number of contacts they have with others — they all require clustering that’s controlled by some sort of parameters. It turns out that if we tweak even one parameter, everything changes wildly.
We believe that can explain another key anomaly. We noticed that during the June 2020 protests following the death of George Floyd, our data didn’t show a spike in anything — it didn’t show more people visiting places, didn’t show more people congregating, didn’t show more contacts between people — it looked like a little flat line. And we have drone photos that show 60,000 people packed in front of the art museum. After a lot of thinking, we arrived at an explanation: if parameter values are fine-tuned to detect visits to things like small businesses, then the clustering algorithm will not catch things happening in large open areas. Similarly, a clustering algorithm that works really well for a user with a lot of pings every hour will not work for a user that has a few pings in a week.
“All the useful metrics we can glean from this data […] require clustering that’s controlled by some sort of parameters. It turns out that if we tweak even one parameter, everything changes wildly.”
Q: What have been some of the most rewarding experiences you’ve had while working on this?
A: The most rewarding part has definitely been working with undergrads, and getting a sense of how much they know. It could be that Penn is such an amazing school, or that Duncan just recruits really good research assistants, but they know so much compared to what I knew when I was an undergrad. They’re amazing, and a huge help. It truly feels like a little industry working with them at the Lab.
The other rewarding thing has been realizing just how many bridges can be built between epidemic modeling and machine learning. Generative neural networks have come a long way and can, for example, complete a picture of your face that’s missing a rectangle, in a creepily accurate way. Building off of that principle, we’re taking this problem — not having a lot of data for many users — and trying to fill in the gaps using a deep networks. Similarly, we are trying to use neural networks to infer the true state of an epidemic using observed caseload data and mobility metrics using generative neural networks, in the same way that a neural network can infer — or imagine — art, given a prompt. Taking all of this interdisciplinary research and making it truly data-driven in this way has definitely been satisfying.
Q: What’s next? Tell me about the future of your projects, or about ways you’re hoping to expand upon your research.
A: We’re wrapping up two papers: one on validating the data, and one on filling in gaps with a neural network. When those are done, we have research planned that actually dives into epidemic modeling. It sounds ambitious, but we’re trying to tackle a few of the biggest obstacles in the field:
First, epidemic modeling has not been as data-driven as it could be. For the most part, when we started, it was rooted in simulations that use synthetic networks and setting parameters to arbitrary values without justification, and could not capture the dynamic nature of human mobility. Second, we want to allow epidemic models to use data-driven networks, and determine the correct way to define and integrate such networks from sources like GPS data, as well as determine which features of these real networks make a difference in epidemic spread. The third part is to come up with a way to verify that a model’s parameters are correct and well-calibrated. As of today, there’s no convincing way of doing that, because the models are too high-dimensional, but we have some ideas that combine machine learning and Bayesian statistics. Finally, we want to crack the problem of designing optimal interventions to curb an epidemic. Trial and error using simulations can provide some informative takeaways, but solving a carefully posed optimization problem could provide very efficient and targeted interventions — something like closing specific venues for specific periods of time depending on the current number of cases.
We might have been able to save a lot of lives, time, and money if we didn’t face these obstacles, but we’re still so far from that that it’s shocking. We know how to do some things — we can hit an asteroid with a little satellite, right in the middle, and change its orbit! — that sound like they should be a lot harder than understanding how an epidemic spreads. And yet, the difficulty in some of the simplest applications of epidemic modeling still seems…exorbitant.
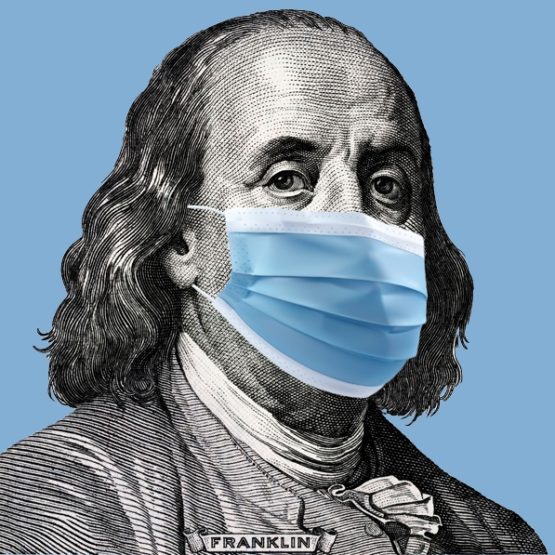
Related: COVID-Philadelphia
The CSSLab is building a collection of interactive data dashboards that visually summarize human mobility patterns over time and space for a number of cities, starting with Philadelphia, along with highlighting potentially relevant demographic correlates.
AUTHORS
EMMA ARSEKIN
—
Communications Specialist