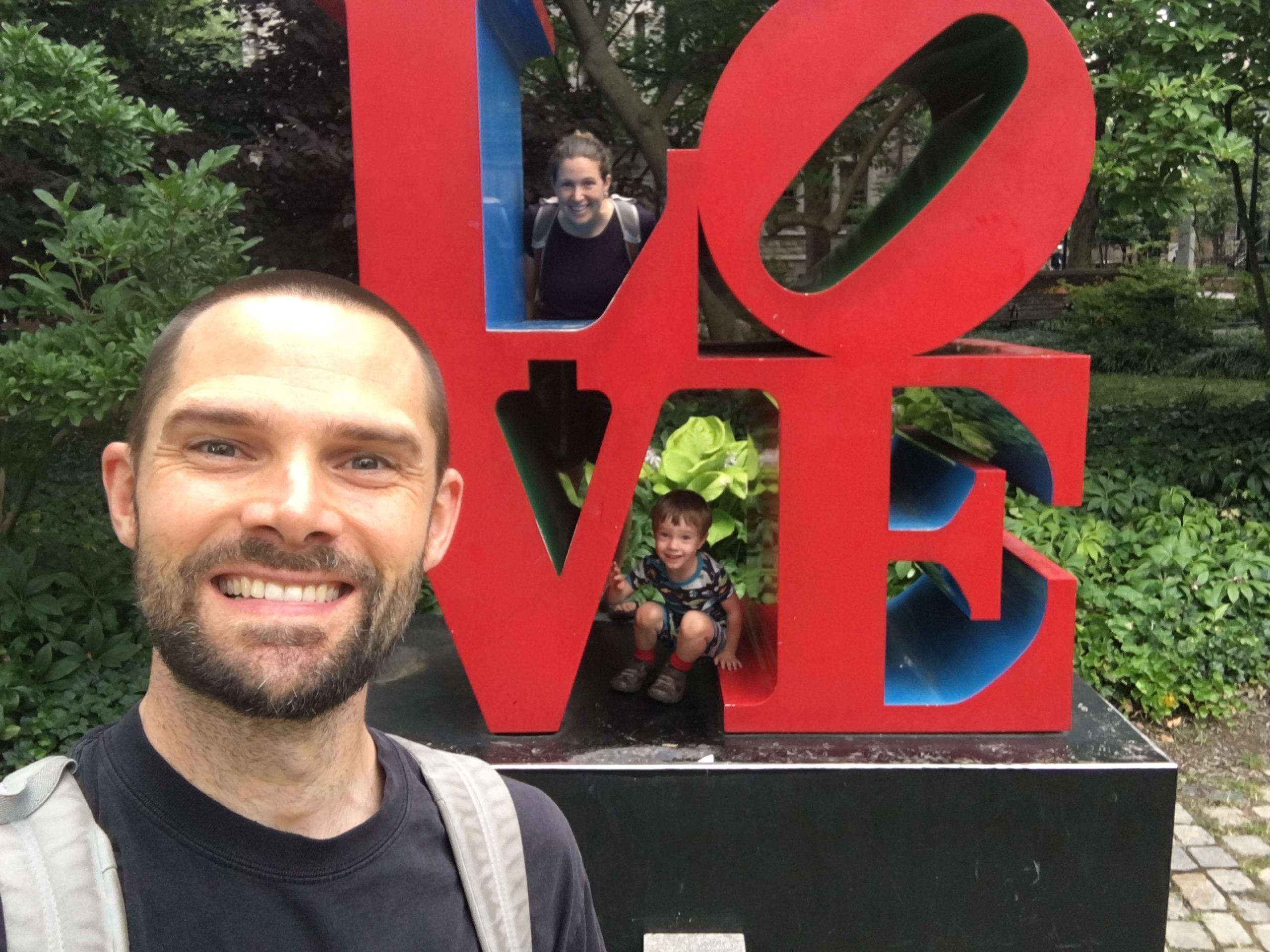
As a key figure in the CSSLab’s work on high-throughput virtual lab experiments, post-doctoral researcher James Houghton aims to refocus social science around large-scale, data-driven insights. In this researcher spotlight, he shares about his path to computational social science research, his work at the CSSLab, and the exciting future for his most recent project on small-group deliberation.
—
Q: Tell me a bit about your background. What did you do before computational social science?
A: I started out as an aerospace engineer. I’ve always had an engineering mindset, and I really enjoy thinking about the ways that all the parts of an engineered system slot together to create some sort of emergent behavior. It’s in stitching together different pieces of functionality that you get something new and interesting.
When I came out of my undergrad, I worked for a few years in a small aerospace R&D company in Kendall Square—right next to my old lab at MIT—where we built interesting prototypes of different aircraft. The technical part of it I really enjoyed; we’d make our models on the computer and say, “ok, this is how we think this aircraft will behave,” and then we’d go out and build a working prototype in the lab. We’d sit there and figure out how to design these things using simulations, and then go and test them, in a really fast cycle between models and experiments.
Q: What changed? Did something draw you away from that line of work?
A: I appreciated the challenge and the complexity of what we were doing, but I started to get uncomfortable with the products we were building. A lot of our contracts were for the Department of Defense, designing aircraft that would be militarized, and that would give power to people who already had lots of power. And maybe there’s a place for that, but I felt like I would rather be working to build a world where we didn’t need those systems.
So I started to look for ways to use complex systems modeling, along with iteration between simulations and experiments, to look at social problems. I wanted to see if we could use the same techniques, the same math, the same approach to learning that we used in the aerospace lab to think about how conflicts start, and how they could be prevented. So eventually I made my way from building engineering systems—where you start with an objective and say, “ok, what pieces do we need to make that happen?”—to social systems, where you don’t have that luxury. You start with the pieces instead, and that’s a lot harder.
“I wanted to see if we could use the same techniques, the same math, the same approach to learning that we used in the aerospace lab to think about how conflicts start, and how they could be prevented.”
That took me back to school. I worked as an RA for a Professor in the business school at MIT for a couple of years, looking at how cultural narratives shape the forms of social conflict. Then I moved over to a Ph.D. in system dynamics, which is essentially what you get when you take a bunch of engineers and have them look at business systems. This field came out of MIT in the 1950’s, when engineers who had been developing computers and feedback controllers for deck guns on Navy ships started to recognize that feedback processes were also major drivers of social systems. Their work spawned a whole methodology for thinking about and simulating social and business systems. I learned about modeling social systems, and about using simulations to craft sociological theory and design experiments with human participants. It was great, and it was hard, and it was fun.
Q: How did you become involved with the CSSLab?
A: In designing my thesis experiment, I started working with Abdullah Almaatouq, a professor at [MIT] Sloan who is an expert in designing online multiplayer experiments. He introduced me to Duncan [Watts] at a conference, and we had a good chat. There was a lot of overlap in the direction that he was hoping to go and the things that I was thinking and doing, and we hit it off. Now I get to work with both of them!
Since then, it’s been a really lovely experience getting to work with the Lab. It’s the first time in a long time that I’ve collaborated so deeply on shared projects, and I’m really enjoying that. There are so many exceptionally talented people here, and it’s such a privilege to be able to work with them. You know, they say you probably shouldn’t stay in a post-doc forever, but I’m really tempted….
Q: You’ve mentioned a few things that you’re working on now at the Lab. Tell me more about those projects.
A: There are two things that I’ve been giving most of my attention to. One is the high-throughput project on team performance, thinking about our data models. These models integrate data from all of the different aspects of our experiment across a huge experiment design space to understand how our results vary across the space. We’ve been thinking about how we can efficiently use information from one point in the design space to make predictions about outcomes at another point in the design space, and to identify the most informative next experiment.
The other project that I’m just starting is a study of small-group deliberation. In some ways, it’s a continuation of my thesis work, looking at how conflict forms in groups. There’s a lot of research that says that when you have a small group deliberating a contentious topic, you should use a particular intervention or structure to help them succeed. But other people come along and say, “well actually, this is the sort of intervention that you want.” Unsurprisingly, the right intervention depends on the type of deliberation, and the type of group, and the context in which they’re deliberating, so you can’t just find one answer—you need to find a map over some large-dimensional space to find out which regions are most supported by one particular intervention and which regions are better supported by some other intervention.
Q: Tell me more about your work on group deliberation. What’s your vision for how it will pan out?
A: We’re working with a huge space. We’re going to have to do thousands of experiments—more than any one lab can do—so we need a way of integrating knowledge from across a lot of different teams. Our team at the Lab will start to build some machinery and infrastructure for conducting deliberation experiments at scale, but we’re only going to get so far with that. The hope is that, eventually, we can invite other researchers working in this space to take advantage of the tools we’ve built and collaborate to create a shared dataset that we can all use. Even further down the road, I’d like to open the platform to participants from the outside world—so a community group could have a meeting using our tools, and they would get the benefit of the best science we have on how to support the needs of their particular deliberation, and we’d get the benefit of learning from their experience. That could really help us get the density of samples we will need to map the space well and be useful to people.
We’re starting with relatively unopinionated models of how different interventions vary across the design space. Our hope is to map how the interventions and the features of the design space relate to each other given the data. But there are a lot of pieces that go into that, in terms of serving the experiments, creating models that can integrate all the data in a way that allows us to make predictions about what we should try next, trying the next high-leverage samples, and constructing the groups that we’d need to do that. We’re always learning and figuring out what we need to study next in order to learn best. In that way, it’s a bit like being back in the aerospace lab; it reminds me of being entirely surrounded by simulations and using our models to think about what we’re doing in the lab and then using the lab to update our models.
We ran our first test last week, and my hope is that we’re going to keep up a weekly development cycle, running tests frequently to learn how to conduct these experiments and how to build infrastructure that works at scale. From there, we’ll slowly build up our cadence so that we’re taking tens to hundreds of samples per week and consistently integrating data and making new predictions.
Q: What sets this project apart from other social science research?
A: Normally, a social scientist might spend a year deciding on which experiment to run based on theoretical assumptions, picking a particular operationalization of their research question, designing a single experiment, collecting the data in a short burst, and then analyzing and publishing it. Our plan is to never really stop taking data, which is a very different approach from how we normally do things in social science research.
For example, on Monday we might pick a set of experiments we want to run that week. We’d then ask research collaborators around the world to predict what the results of those experiments will be. Then, after running experiments Monday through Friday, we’ll take our data and ask, “ok, whose model did the best?” We can then score the predictions of various theoretical and atheoretical models against the actual data, and release the new batch of data to allow researchers to use it to train their models for the next week. And we’d use the results of those models to identify the best samples for the next set of data collection, and the next week do it all over again, creating a continuous process of learning and integrating data.
“Our plan is to never really stop taking data, which is a very different approach from how we normally do things in social science research.”
So instead of the typical cycle where the design of the experiment through the publication of data takes a year or two, we’re going to try to do that every week, which is a totally different way of thinking about social science. It forces us to think about the assumptions that go into research, and which constraints of research are due to good research practices and which are due to the limitations of our thinking and technology. When we can build new tools and new approaches, but still maintain or enhance the academic rigor of what we’re doing—that’s when we win.
Q: What are some of the biggest challenges you’ve run into so far in your work?
A: One of the hardest things, technically, is dealing with this enormous high-dimensional space. Every time you add a dimension, you’re exponentially increasing the number of samples you need—it’s the curse of dimensionality. We’re starting with dimensions that, based on the literature, people already think are really important, and we’ll study them by varying the parameters across those dimensions. But we’ll measure a bunch of other stuff. I might vary the size of my deliberative group, along with the expected contentiousness of the topic, but I’ll also measure participants’ average age, or their age diversity, or a number of other features.
What you get at that point is a two-dimensional space that plots complexity and group size, and is a flattened projection of all these other dimensions that we’ve measured. So one of the roles of the models will be to ask, “of all the dimensions that I’ve measured or conceived, which of them, given the data that I have, are the most likely to be worth exploring?” This is a form of interactive feature selection—not just asking what features in the existing literature might be important to include in our models, but what features in the data we want to practically vary in ongoing experiments. We can then add dimensions and explore them intentionally.
This is interesting because, in experiments with human beings, there’s a cost for every measurement. A lot of the things that I can measure require me to ask questions of the participants—how old are you, what’s your income bracket, etc.—and participants can only realistically answer so many questions. So if I choose a dimension that’s uninformative, and I’m spending some of my “attention budget” for the participant to collect that information, then I’m basically wasting time and samples. Figuring out how to balance these competing needs of measuring the dimensions, controlling the size of the dimension space, and managing participants’ attention is really hard.
Q: What’s in store for your projects?
A: I’m really excited to have a whole cohort of students coming on for the summer to help build this infrastructure. I don’t know if they really know what they’re in for yet, but pushing them to run one experiment per week and have some sort of substantive development as we go through is going to be a lot of work. It’ll be a high standard to live up to, and I’m excited about the energy that that cadence brings. The ability to have this team of people all working together to achieve these objectives and having a really rigorous set of deadlines, like when to launch with human participants, is a different sort of energy than we usually have over the summer in academia. I’m really looking forward to it.
Learn more about James’ current research by visiting our Group Dynamics project page.
AUTHORS
EMMA ARSEKIN
—
Communications Specialist