Research
Penn Media Accountability Project (PennMAP)
PennMAP is building technology to detect patterns of bias and misinformation in media from across the political spectrum and spanning television, ratio, social media, and the broader web. We will also track consumption of information via television, desktop computers, and mobile devices, as well as its effects on individual and collective beliefs and understanding. In collaboration with our data partners, we are also building a scaleable data infrastructure to ingest, process, and analyze tens of terabytes of television, radio, and web content, as well as representative panels of roughly 100,000 media consumers over several years.
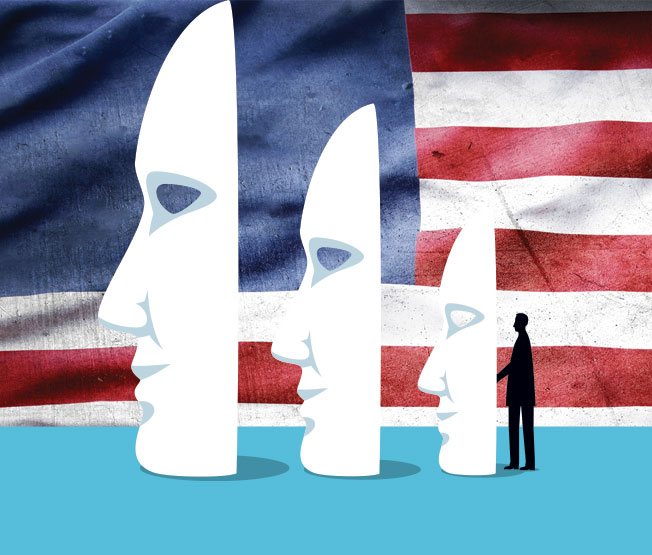
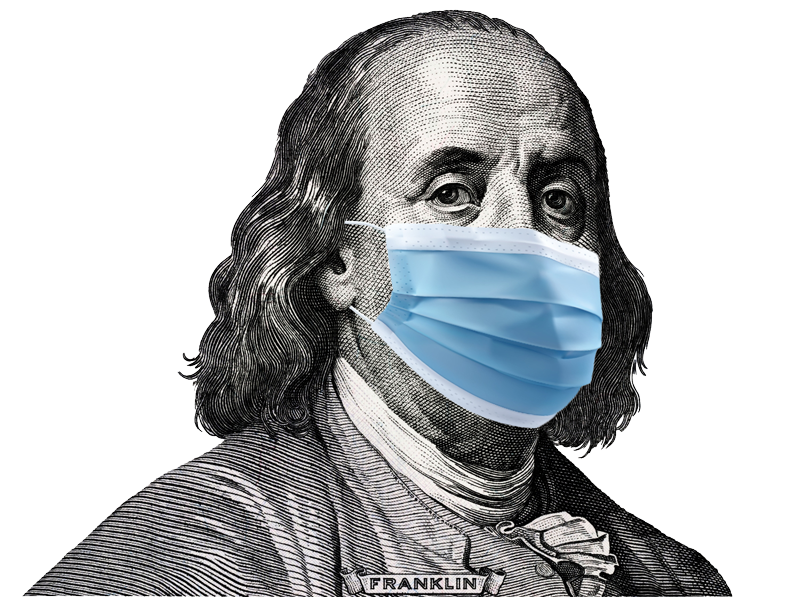
COVID – Philadelphia
Our team is building a collection of interactive data dashboards that visually summarize human mobility patterns over time and space for a collection of cities, starting with Philadelphia, along with highlighting potentially relevant demographic correlates. We are estimating a series of statistical models to identify correlations between demographic and human mobility data (e.g. does age, race, gender, income level predict social distancing metrics?) and are using mobility and demographic data to train epidemiological models designed to predict the impact of policies around reopening and vaccination.
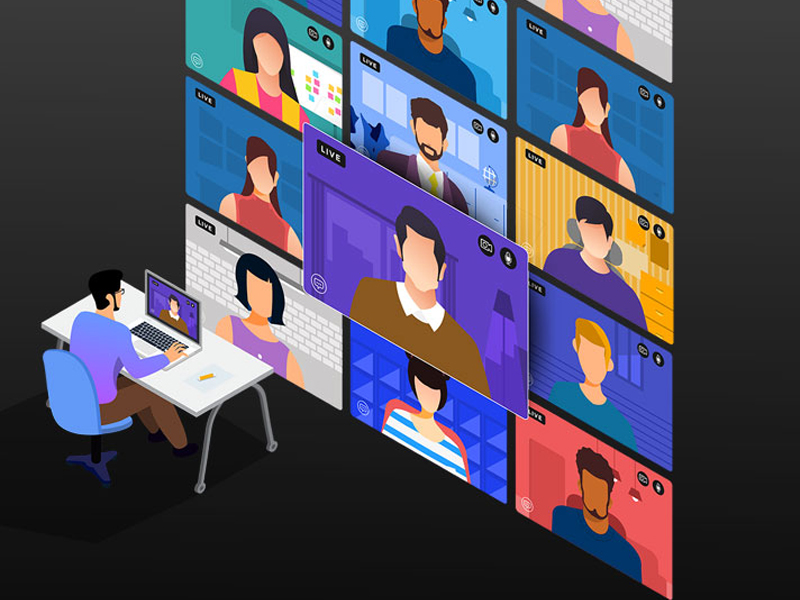
High-Throughput Experiments on Group Dynamics
To achieve replicable, generalizable, scalable, and ultimately useful social science, we believe that is necessary to rethink the fundamental “one at a time” paradigm of experimental social and behavioral science. In its place we intend to design and run “high-throughput” experiments that are radically different in scale and scope from the traditional model. This approach opens the door to new experimental insights, as well as new approaches to theory building.
Common Sense
This project tackles the definitional conundrum of common sense head-on via a massive online survey experiment. Participants are asked to rate thousands of statements, spanning a wide range of knowledge domains, in terms of both their own agreement with the statement and their belief about the agreement of others. Our team has developed novel methods to extract statements from several diverse sources, including appearances in mass media, non-fiction books, and political campaign emails, as well as statements elicited from human respondents and generated by AI systems. We have also developed new taxonomies to classify statements by domain and type.
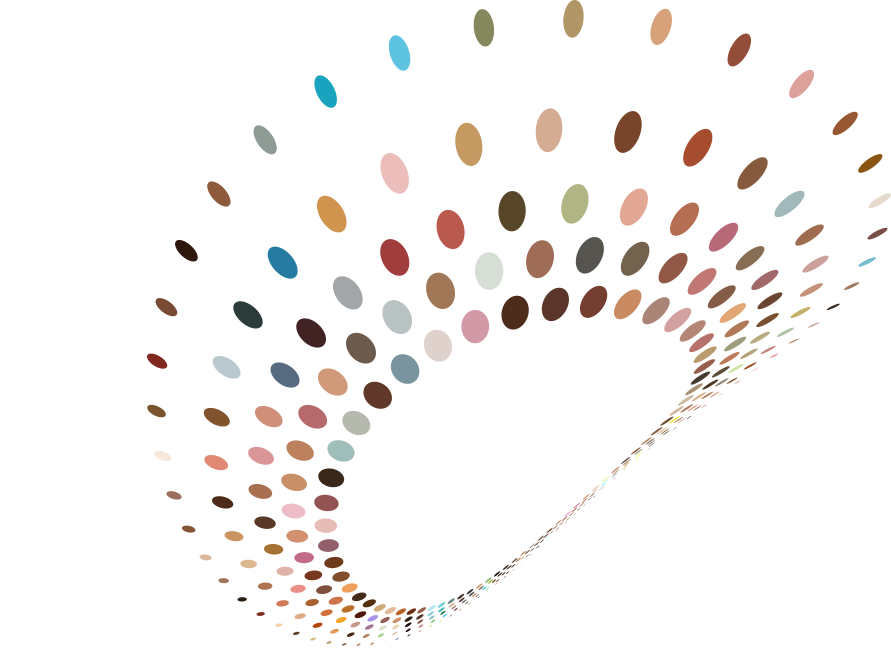
News
Violent Language in Films Has Increased Since the 1970s: A New Study
Violent entertainment has made it into the public discourse due to rising concerns about the graphic nature of highly popular video game franchises including Grand Theft Auto (GTA) and Call of Duty. But what about violence in films which are enjoyed by a much larger and more diverse audience? After the R Rating was established by the Motion Picture Association of America (MPAA) film rating system in 1968, there was an increase in violent content in films thereafter.
Research Assistant Spotlight: Priya D’Costa Presents at NeurIPS
This past weekend CSSLab alumna Priya DCosta presented a poster titled What do you say or how do you say it? at NeurIPS 2024 Behavioral ML Workshop, a first-time workshop on exploring the incorporation of insights from the behavioral sciences into AI models/systems in Vancouver on December 10-15. In this RA spotlight, Priya shares more on her background and research leading up to NeurIPS.
Grumpy Voters Want Better Stories. Not Statistics
In the final count, Trump collected 312 electoral votes to 226 for Democratic nominee Kamala Harris. While some votes are still being counted, the broad trends that won the election for Trump are also coming into focus. Echoing public opinion scholars, Duncan Watts of the University of Pennsylvania’s Annenberg School for Communication, author of Everything Is Obvious Once You Know the Answer, believes that Trump benefited from a broad anti-incumbent trend seen in elections worldwide; that sentiment swung enough undecided voters to his tally to win him the swing states needed for victory.