Opening the black box of groups and team’s communication dynamics
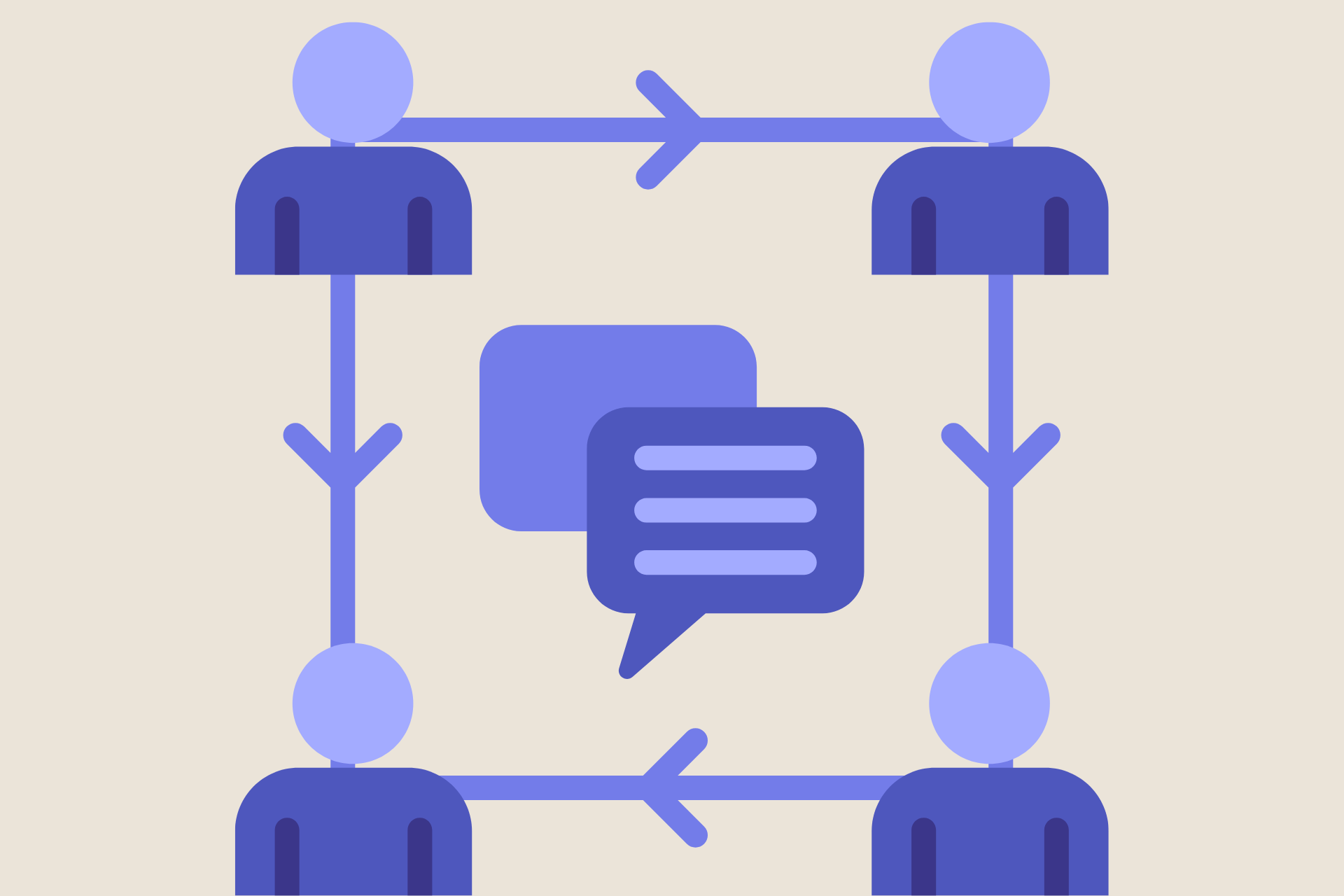
Team Communication
How do successful teams talk? When are deep-seated disagreements resolved constructively? Why do conversations help players become cooperative in economic games? Our research applies computational tools — such as cutting edge natural language process (NLP) and machine learning methods — to unpack how and why group conversations “succeed.”
Effective communication is often cited as a critical attribute of a high-performing team (Marlow et al., 2018; Marks et al., 2001). Businesses widely promote and invest in communication skills: articles with tips for improving team communication abound (Gallo, 2022; Jolaoso, 2023; Tawakol, 2018), and firms conduct workshops costing upwards of $6,500 per day (ECG, 2023).
Given this immense interest in effective team communication, it may be surprising to observe that, in fact, there is little consensus on what “effective” means or how to measure it. For example, “communication quality” has been variously defined as the willingness to share ideas (B. H. Bradley et al., 2013); timeliness of the message (Kashive et al., 2022; González-Romá & Hernández, 2014); perceived appropriateness, openness, and richness (Lam, 2015); and the positivity of message sentiment (Pöysä-Tarhonen et al., 2016). While everyone seems to agree that quality communication is important, no one seems to agree on what quality communication is.
This example reveals two related challenges in the study of team communication. The first is a challenge of definition. While many features have been proposed to characterize effective communication, they form only a loose collection of constructs rather than a coherent definition of communication effectiveness. The second challenge is with measurement. Even with a consistent definition of effective communication, theoretically-important features do not always map onto specific, measurable behaviors; two instances of perceived “open” or “clear” communication may not refer to the same underlying activity. Moreover, many constructs rely on self-reported measures (Marlow et al., 2018), which can be subject to various forms of bias, such as social desirability (Viney, 1983) and outcome bias (Staw, 1975; Rosenzweig, 2014). This loose coupling between communication measurements and concrete behaviors makes it difficult to translate findings from generalities (“it’s important to be clear”) to actionable improvements.
Our research aims to tackle both of these challenges head-on. First, our ongoing work explores the relevant attributes and dynamics of conversation in a variety of settings, from negotiations to social media websites to online multi-player games. In each setting, we develop models that help us unpack the “black box” of conversation dynamics, exploring how patterns of “more effective” conversations (negotiations that end in a mutually-satisfactory deal; debates in which someone changes their point of view; public goods games in which the members choose to cooperate rather than defect) differ from “less effective” conversations (negotiations that fail to reach a satisfactory deal; debates that end in name-calling; public goods games that devolve into defection). Second, we are building an open-source toolkit (https://teamcommtools.seas.upenn.edu/) for measuring team communication across many settings. Our python package, team_comm_tools, is freely available for download, and extracts more than 160 features from an open-ended text conversation in a matter of minutes. By extracting, structuring, and implementing attributes of conversations from the academic literature, we hope to create a repository that helps researchers rapidly bootstrap analyses, explore a range of options for measuring constructs of interest, and access a multitude of possible analyses right out of the box.
PEOPLE
(Xinlan) Emily Hu
Yuxuan Zhang
—
Data Scientist
Shruti Agarwal
—
Undergraduate Student Researcher
Pradnaya Pathak
—
Undergraduate Student Researcher
Amy Zheng
—
Undergraduate Student Researcher
Priya DCosta
—
Masters’s Alumna
Yashveer Singh Sohi
—
Masters’s Alumnus
James Houghton
—
Research Scientist
Additional Collaborators
Abdullah Almaatouq (MIT Sloan School of Management)
Jared Curhan (MIT Sloan School of Management)
Mohammed Alsobay (MIT Sloan School of Management)
Burint Bevis (Imperial College London)
Mark Kennedy (Imperial College London)
FEATURED PUBLICATIONS
The Effects of Group Composition and Dynamics on Collective Performance Journal Article
In: Topics in Cognitive Science, 2023.
Task complexity moderates group synergy Journal Article
In: Proceedings of the National Academy of Sciences, vol. 118, no. 36, 2021.
Scaling up experimental social, behavioral, and economic science Technical Report
2021.
My Team Will Go On: Differentiating High and Low Viability Teams through Team Interaction Journal Article
In: Proceedings of the ACM Human-Computer Interaction, vol. 4, no. CSCW3, pp. 1-27, 2021.
Empirica: a virtual lab for high-throughput macro-level experiments Journal Article
In: Behavior Research Methods, pp. 1–14, 2021.
Can We Just Start Over Again? Resetting Remote Team Dynamics Journal Article
In: 2020.
Parallel Worlds: Repeated Initializations of the Same Team To Improve Team Viability Journal Article
In: Proceedings of the ACM Human-Computer Interaction, vol. 4, no. CSCW1, pp. 22, 2020.
Related
CSSLab Establishes Virtual Deliberation Lab to Reduce Affective Polarization
When a Republican and a Democrat sit down to discuss gun control, how is it going to go? Conversations between Republicans and Democrats can be either productive or polarizing and social scientists want to understand what makes conversations between people from competing social groups succeed, as positive conversations have proven to be one of the most effective ways to reduce intergroup conflict. However, when conversations go poorly, they can instead increase polarization and reinforce negative biases.
The mechanics of collaboration
Like many clichés, the origins of the common mantra “Teamwork makes the dream work” is rooted in a shared experience.
For Xinlan Emily Hu, a fourth-year Ph.D. student at Wharton, that is, however, more than just a catchy saying. It is the foundation of her research into the science of teamwork. As she puts it, “The magic of a successful team isn’t just in having the right people; it’s in how those people interact and communicate.”
The Team Communication Toolkit: Emily Hu’s Award-Winning Project
Emily Hu, a fourth year Wharton Operations, Information, and Decisions PhD student at the Computational Social Science Lab (CSSLab), has just launched her award-winning Team Communication Toolkit at the Academy of Management Conference on August 12 in Chicago. This toolkit allows researchers to analyze text-based communication data among groups and teams by providing over a hundred research-backed conversational features, eliminating the need to compute these features from scratch.
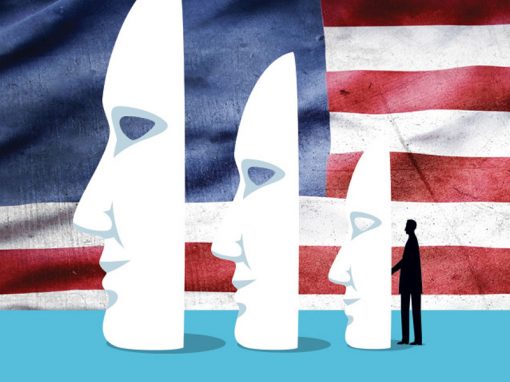
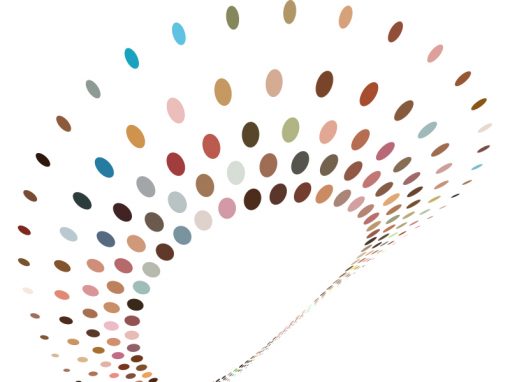
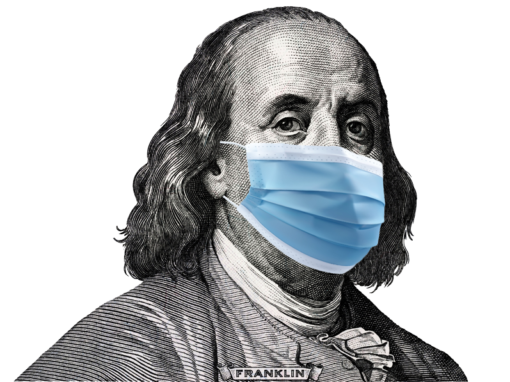
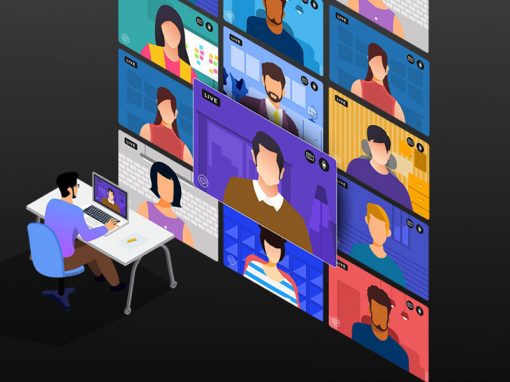
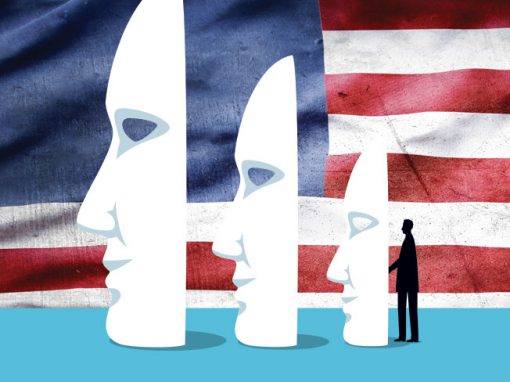