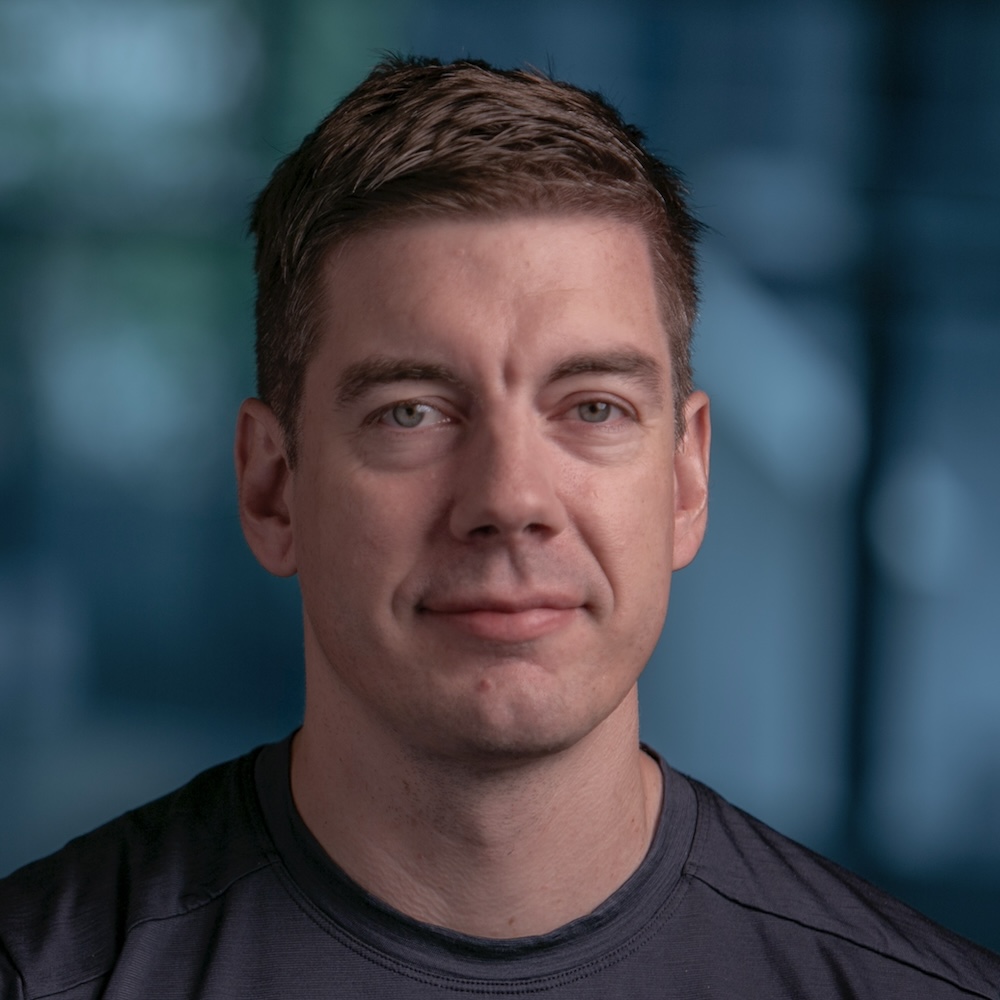
Researcher
Mark Whiting
Senior Computational Social Scientist
Mark Whiting is a Senior Computational Social Scientist at the CSSLab, affiliated with the Department of Computer & Information Science at Penn Engineering and the Department of Applied Science and Operations, Information and Decisions at Wharton. He builds systems to study how people behave and coordinate at scale. At the Lab, he leads the High-Throughput Experiments on Group Dynamics, COVID-Philadelphia, and Common Sense Projects.
Mark was previously a post-doctoral researcher under Michael S. Bernstein in the HCI group in Computer Science at Stanford. He holds bachelor’s and master’s degrees in Industrial Design from RMIT and KAIST respectively, and a PhD in Mechanical Engineering from CMU.
Publications +
Nguyen, Tuan Dung; Watts, Duncan J.; Whiting, Mark E.
Empirically evaluating commonsense intelligence in large language models with large-scale human judgments Working paper
2025.
@workingpaper{nokey,
title = {Empirically evaluating commonsense intelligence in large language models with large-scale human judgments},
author = {Nguyen, Tuan Dung and Watts, Duncan J. and Whiting, Mark E. },
url = {https://arxiv.org/abs/2505.10309},
doi = { https://doi.org/10.48550/arXiv.2505.10309},
year = {2025},
date = {2025-05-22},
abstract = {Commonsense intelligence in machines is often assessed by static benchmarks that compare a model's output against human-prescribed correct labels. An important, albeit implicit, assumption of these labels is that they accurately capture what any human would think, effectively treating human common sense as homogeneous. However, recent empirical work has shown that humans vary enormously in what they consider commonsensical; thus what appears self-evident to one benchmark designer may not be so to another. Here, we propose a novel method for evaluating common sense in artificial intelligence (AI), specifically in large language models (LLMs), that incorporates empirically observed heterogeneity among humans by measuring the correspondence between a model's judgment and that of a human population. We first find that, when treated as independent survey respondents, most LLMs remain below the human median in their individual commonsense competence. Second, when used as simulators of a hypothetical population, LLMs correlate with real humans only modestly in the extent to which they agree on the same set of statements. In both cases, smaller, open-weight models are surprisingly more competitive than larger, proprietary frontier models. Our evaluation framework, which ties commonsense intelligence to its cultural basis, contributes to the growing call for adapting AI models to human collectivities that possess different, often incompatible, social stocks of knowledge.},
keywords = {},
pubstate = {published},
tppubtype = {workingpaper}
}
Whiting, Mark E.; Watts, Duncan J.
A framework for quantifying individual and collective common sense Journal Article
In: PNAS, vol. 121, iss. 4, 2024.
@article{nokey,
title = {A framework for quantifying individual and collective common sense },
author = {Whiting, Mark E. and Watts, Duncan J. },
doi = {https://doi.org/10.1073/pnas.2309535121},
year = {2024},
date = {2024-01-16},
urldate = {2024-01-16},
journal = {PNAS},
volume = {121},
issue = {4},
abstract = {The notion of common sense is invoked so frequently in contexts as diverse as everyday
conversation, political debates, and evaluations of artificial intelligence that its meaning might
be surmised to be unproblematic. Surprisingly, however, neither the intrinsic properties of
common sense knowledge (what makes a claim commonsensical) nor the degree to which it
is shared by people (it’s “commonness”) have been characterized empirically. In this paper,
we introduce an analytical framework for quantifying both these elements of common sense.
First, we define the commonsensicality of individual claims and people in terms of latter’s
propensity to agree on the former and their awareness of one another’s agreement. Second,
we formalize the commonness of common sense as a clique detection problem on a bipartite
belief graph of people and claims, defining pq common sense as the fraction q of claims shared
by a fraction p of people. Evaluating our framework on a dataset of 2,046 raters evaluating
4,407 diverse claims, we find that commonsensicality aligns most closely with plainly-worded,
fact-like statements about everyday physical reality. Psychometric attributes such as social
perceptiveness influence individual common sense, but surprisingly demographic factors
such as age or gender do not. Finally, we find that collective common sense is rare: at most a
small fraction p of people agree on more than a small fraction q of claims. Together, these
results undercut universalistic beliefs about common sense but also open new questions
about its variability of that are relevant both to human and artificial intelligence. },
keywords = {},
pubstate = {published},
tppubtype = {article}
}
conversation, political debates, and evaluations of artificial intelligence that its meaning might
be surmised to be unproblematic. Surprisingly, however, neither the intrinsic properties of
common sense knowledge (what makes a claim commonsensical) nor the degree to which it
is shared by people (it’s “commonness”) have been characterized empirically. In this paper,
we introduce an analytical framework for quantifying both these elements of common sense.
First, we define the commonsensicality of individual claims and people in terms of latter’s
propensity to agree on the former and their awareness of one another’s agreement. Second,
we formalize the commonness of common sense as a clique detection problem on a bipartite
belief graph of people and claims, defining pq common sense as the fraction q of claims shared
by a fraction p of people. Evaluating our framework on a dataset of 2,046 raters evaluating
4,407 diverse claims, we find that commonsensicality aligns most closely with plainly-worded,
fact-like statements about everyday physical reality. Psychometric attributes such as social
perceptiveness influence individual common sense, but surprisingly demographic factors
such as age or gender do not. Finally, we find that collective common sense is rare: at most a
small fraction p of people agree on more than a small fraction q of claims. Together, these
results undercut universalistic beliefs about common sense but also open new questions
about its variability of that are relevant both to human and artificial intelligence.
Almaatouq, Abdullah; Griffiths, Thomas L.; Suchow, Jordan W.; Whiting, Mark E.; Evans, James; Watts, Duncan J.
Beyond Playing 20 Questions with Nature: Integrative Experiment Design in the Social and Behavioral Sciences Journal Article
In: Behavioral and Brain Sciences, 2022.
@article{nokeyb,
title = {Beyond Playing 20 Questions with Nature: Integrative Experiment Design in the Social and Behavioral Sciences },
author = {Almaatouq, Abdullah and Griffiths, Thomas L. and Suchow, Jordan W. and Whiting, Mark E. and Evans, James and Watts, Duncan J. },
url = {https://www.cambridge.org/core/journals/behavioral-and-brain-sciences/article/abs/beyond-playing-20-questions-with-nature-integrative-experiment-design-in-the-social-and-behavioral-sciences/7E0D34D5AE2EFB9C0902414C23E0C292#article},
doi = {10.1017/S0140525X22002874},
year = {2022},
date = {2022-12-21},
urldate = {2022-12-21},
journal = {Behavioral and Brain Sciences},
abstract = {The dominant paradigm of experiments in the social and behavioral sciences views an experiment as a test of a theory, where the theory is assumed to generalize beyond the experiment's specific conditions. According to this view, which Alan Newell once characterized as “playing twenty questions with nature,” theory is advanced one experiment at a time, and the integration of disparate findings is assumed to happen via the scientific publishing process. In this article, we argue that the process of integration is at best inefficient, and at worst it does not, in fact, occur. We further show that the challenge of integration cannot be adequately addressed by recently proposed reforms that focus on the reliability and replicability of individual findings, nor simply by conducting more or larger experiments. Rather, the problem arises from the imprecise nature of social and behavioral theories and, consequently, a lack of commensurability across experiments conducted under different conditions. Therefore, researchers must fundamentally rethink how they design experiments and how the experiments relate to theory. We specifically describe an alternative framework, integrative experiment design, which intrinsically promotes commensurability and continuous integration of knowledge. In this paradigm, researchers explicitly map the design space of possible experiments associated with a given research question, embracing many potentially relevant theories rather than focusing on just one. The researchers then iteratively generate theories and test them with experiments explicitly sampled from the design space, allowing results to be integrated across experiments. Given recent methodological and technological developments, we conclude that this approach is feasible and would generate more-reliable, more-cumulative empirical and theoretical knowledge than the current paradigm—and with far greater efficiency.},
keywords = {},
pubstate = {published},
tppubtype = {article}
}
Almaatouq, Abdullah; Becker, Joshua; Bernstein, Michael S.; Botto, Robert; Bradlow, Eric T.; Damer, Ekaterina; Duckworth, Angela; Griffiths, Tom; Hartshorne, Joshua K.; Lazer, David; Law, Edith; Liu, Min; Matias, J. Nathan; Rand, David; Salganik, Matthew; Emma Satlof-Bedrick, Maurice Schweitzer; Shirado, Hirokazu; Suchow, Jordan W.; Suri, Siddharth; Tsvetkova, Milena; Watts, Duncan J.; Whiting, Mark E.; Yin., Ming
Scaling up experimental social, behavioral, and economic science Technical Report
2021.
@techreport{almaatouq2021scaling,
title = {Scaling up experimental social, behavioral, and economic science},
author = {Abdullah Almaatouq and Joshua Becker and Michael S. Bernstein and Robert Botto and Eric T. Bradlow and Ekaterina Damer and Angela Duckworth and Tom Griffiths and Joshua K. Hartshorne and David Lazer and Edith Law and Min Liu and J. Nathan Matias and David Rand and Matthew Salganik and Emma Satlof-Bedrick, Maurice Schweitzer and Hirokazu Shirado and Jordan W. Suchow and Siddharth Suri and Milena Tsvetkova and Duncan J. Watts and Mark E. Whiting and Ming Yin.},
url = {https://drive.google.com/file/d/1-4kcD8yn4dTikxrbbm5oaGnaEvj3XQ5B/view?usp=sharing},
doi = {10.17605/OSF.IO/KNVJS},
year = {2021},
date = {2021-06-29},
urldate = {2021-06-29},
pages = {40},
abstract = {The standard experimental paradigm in the social, behavioral, and economic sciences is extremely limited. Although recent advances in digital technologies and crowdsourcing services allow individual experiments to be deployed and run faster than in traditional physical labs, a majority of experiments still focus on one-off results that do not generalize easily to real-world contexts or even to other variations of the same experiment. As a result, there exist few universally acknowledged findings, and even those are occasionally overturned by new data. We argue that to achieve replicable, generalizable, scalable and ultimately useful social and behavioral science, a fundamental rethinking of the model of virtual-laboratory style experiments is required. Not only is it possible to design and run experiments that are radically different in scale and scope than was possible in an era of physical labs; this ability allows us to ask fundamentally different types of questions than have been asked historically of lab studies. We argue, however, that taking full advantage of this new and exciting potential will require four major changes to the infrastructure, methodology, and culture of experimental science: (1) significant investments in software design and participant recruitment, (2) innovations in experimental design and analysis of experimental data, (3) adoption of new models of collaboration, and (4) a new understanding of the nature and role of theory in experimental social and behavioral science. We conclude that the path we outline, although ambitious, is well within the power of current technology and has the potential to facilitate a new class of scientific advances in social, behavioral and economic studies.},
keywords = {},
pubstate = {published},
tppubtype = {techreport}
}