The CSSLab’s Research Data Engineer, Yingquan Li, offers his perspective on data-driven social science and the challenges facing the new field of CSS.
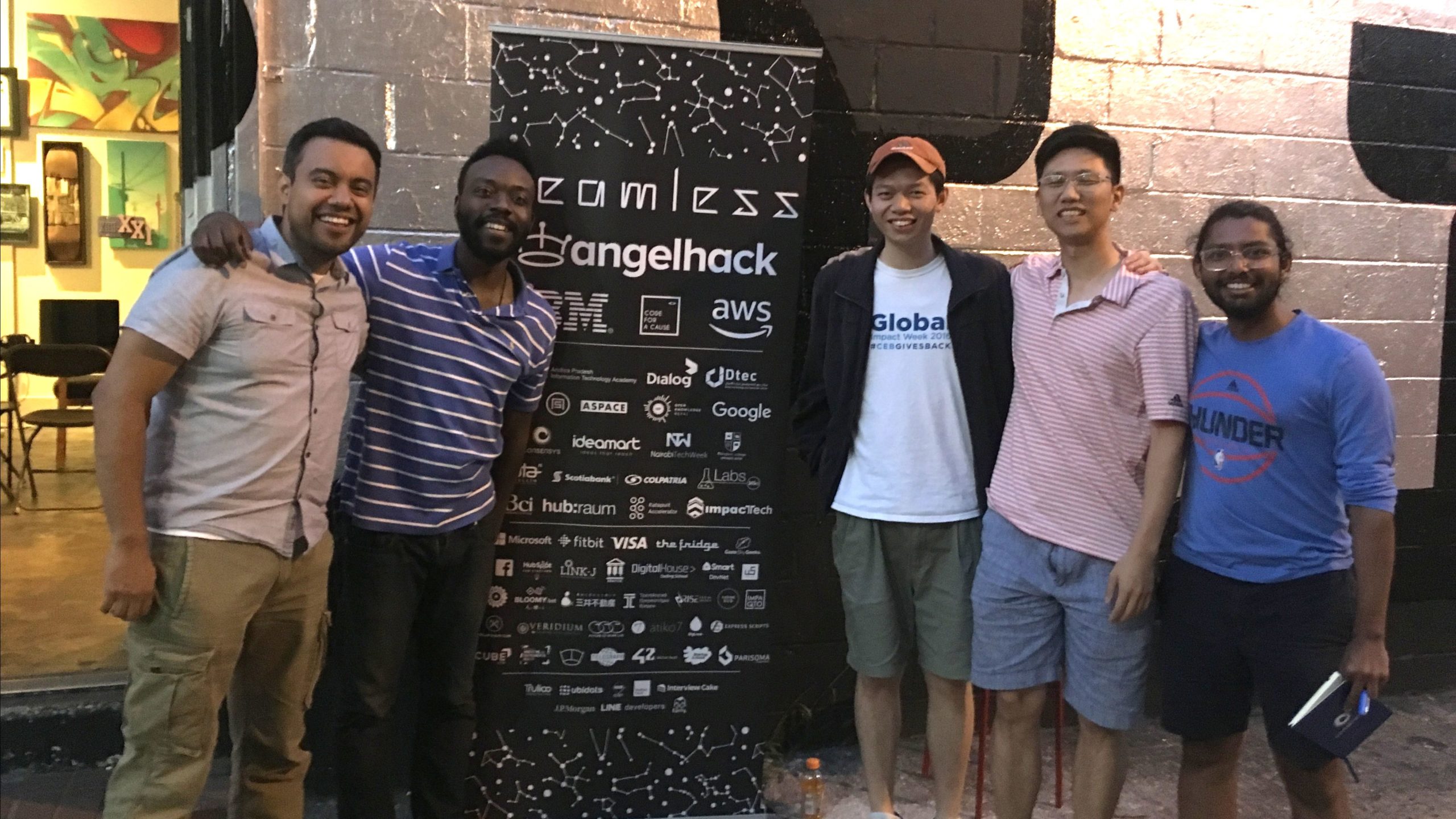
ABOVE: Yingquan Li (third from right), the CSSLab’s Research Data Engineer, at an AWS-sponsored event.
In its first year, the CSSLab has seen its work published in major journals, enjoyed generous financial and material support, and procured an expanding staff and research team. Behind the scenes of these achievements, however, much of the Lab’s learning and growth has taken place when tackling logistical and scaling challenges—perhaps most notably, the challenge of creating a bespoke research infrastructure capable of processing, storing, and analyzing tens of terabytes of data.
Since coming onboard as Research Data Engineer in June 2021, Yingquan Li has worked closely with the CSSLab’s researchers to develop this infrastructure. He has been instrumental in gathering article data from PeakMetrics through one of the Lab’s data providers, Harmony Labs. Between June and October, he also monitored a sync pipeline in the process of transferring 35TB of TVEyes data, and currently helps to parse the Lab’s Nielsen panel data. “I do a little bit of everything,” Yingquan notes, “and through that, you come to realize that our main problems are still being defined.”
“The CSSLab is unique in that our ‘product’ is research and we inhabit this academic space, but the data we’re using is on an industrial scale, and the tools we’re using are industry tools.”
– Yingquan Li
When asked to give insight into the Lab’s major challenges, Yingquan begins at the conceptual level. “The CSSLab is unique in that our ‘product’ is research and we inhabit this academic space,” he explains, “but the data we’re using is on an industrial scale, and the tools we’re using are industry tools.” In his view, bridging the gap between academia and industry has proven to be one of the most interesting challenges the Lab has faced, since he and the Lab’s researchers have come to understand that their academic perspective and practices cannot be cleanly mapped onto what is used in industry. As a result, they have had to adapt new practices and tools to be able to apply their expertise to their specific research pipelines.
“The way we think about using our technology has to be within the context of our research perspective,” Yingquan clarifies, “but innovation comes from figuring out how to frame our work so that all parties understand what’s going on.” The importance of this framing made itself apparent in late 2021, when the Lab realized that one of its data partners was using data sampling and processing practices that, while appropriate for industry use, did not meet the standards necessary for academic research. This discrepancy in expectations caused the Lab to branch out to alternative sources, with Yingquan playing a major role in monitoring and developing the Lab’s new data pipeline.
Before joining the CSSLab, Yingquan earned seven years of experience working in both the private and public sectors. “I had to unlearn a lot of things I learned working in government and industry,” he admits, but appreciates having learned the importance of thinking on your feet when it comes to working with data. He stresses that it is crucial for both seasoned incumbents and newcomers in the field to “not be so enamored with how things were done previously, or with what [they]’re comfortable with,” and instead commit to new frameworks and ways of thinking.
Despite having to adapt to working in an academic research setting, Yingquan sees parallels between work in the Lab and his experiences in industry. He notes that, in many ways, the CSSLab “feels like an early-stage startup” due to the remarkable pace of its research and organizational development during its first year. Drawing from his experience, he advises that, for such a young organization in a comparably young field, it is important to focus on solving one small problem at a time. This helps the team to build the confidence necessary for solving progressively larger problems. “In this kind of environment,” he elaborates, “unpredictability, things not going right, and meandering for a while before you come to the solution are all normal. But over time, you’ll look back and realize that you’ve done a lot more than you think.”
When asked about the advice he’d give to similar labs embarking upon data-driven social science research, Yingquan highlights automation and organizational diligence as key pillars of an effective research team. “Long-term perspective is important, automating things is important, and thinking things through and trying to eliminate technical debt as much as possible upfront is important,” he advises. These practices, he states, should always be supplemented by vision: “Ultimately, the goal is to build a data product that helps people and is genuinely useful to people.”
AUTHORS
EMMA ARSEKIN
—
Communications Specialist