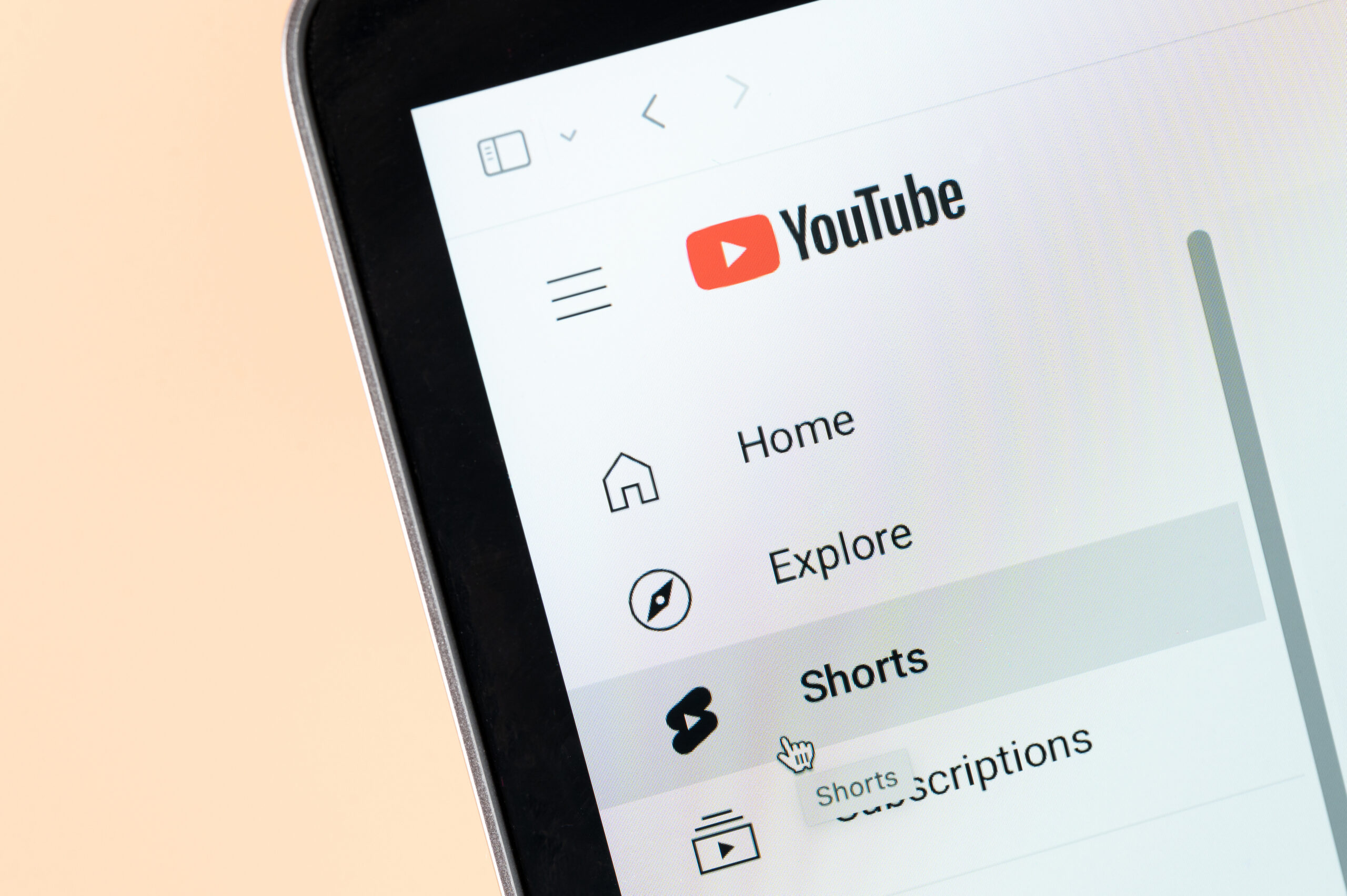
Given the sheer amount of content produced every day on a platform as large as YouTube, which hosts over 14 billion videos, the need for some sort of algorithmic curation is inevitable. As YouTube has attracted millions of views on partisan videos of a conspiratorial or radical nature, observers speculate that the platform’s algorithm unintentionally radicalizes its users by recommending hyperpartisan content based on their viewing history.
But is the algorithm the primary force driving these consumption patterns, or is something else at play?
Researchers at the Computational Social Science Lab (CSSLab) found that hyperpartisan content consumption is primarily driven by user preferences, as when a user’s preferences are not taken into account when selecting what to watch next from a range of recommended videos, the user has a more moderate experience even if they have a history of extreme content consumption.
In their new paper, Homa Hosseinmardi and coauthors Amir Ghasemian, Miguel Rivera-Lanas, Manoel Horta Ribeiro, Robert West, and Duncan J. Watts from the CSSLab introduce a novel experimental method called “counterfactual bots.”
These bots are programmed to simulate what a real user would see if they were to only follow algorithmic recommendations, allowing the authors to see the differences between what is being recommended to the user and what users are actually consuming.
Bot-Powered Experiments
First, all the bots mimicked “real” user behavior by watching the same video sequence so that the YouTube algorithm could learn the user’s preferences. Then, one “control” bot continued on the real user’s path, and the other three “counterfactual” bots switched to relying exclusively on recommendations. The bots programmed to follow rule-based recommendations consumed less partisan content than the bots following the real users; those who consumed very high levels of hyperpartisan content experienced a substantial moderating effect.
In a previous study, Hosseinmardi and her co-authors found that viewers who consumed highly partisan content in “bursts” were more likely to consume more extreme content in the future. But, it remained unclear if this was caused by endogenous user preferences or if it was the exogenous recommender. Revisiting this question with their new methodology, they found that the bots that followed the algorithm’s recommendations consumed less partisan content than their corresponding real user, reflecting that the observed increase is in part due to a change in user preferences towards more hyperpartisan videos.
When the authors found that the algorithm has a moderating effect, they consequently asked: how long does it take for the algorithm to exert this effect? They measured this by quantifying the number of videos the bots watched until extreme content disappeared from their recommendation page. This was done by having bots initially watch video sequences from heavy consumers of far-right content and switch to moderate content. After about 30 videos, the algorithm recommended moderate content, but this moderation took longer for content characterized by higher degrees of partisanship, regardless of political leaning.
Rethinking YouTube Consumption
The authors took a deep dive into the YouTube algorithm and the rabbit holes some viewers find themselves in. With the implementation of counterfactual bots, they were able to compare the the partisanship of algorithm recommendations to the partisanship of real users’ consumption patterns on YouTube, with user consumption reflecting higher partisan scores.
While YouTube is a medium through which hyperpartisan content is readily available, there is a responsibility that lies in users and what they choose to consume. This study is unique from previous literature in that it implements bots that mimic real users and then are instructed to follow parallel paths of both a real user and algorithmic viewership to yield more insights on the algorithm’s role in hyperpartisan consumption, leading to the discovery that the role of the algorithm isn’t as influential as previously thought.
Additionally, the findings of this study challenge the assumption that if one watches extreme content, the algorithm will continue to recommend more of such content, even in cases where the users change their viewing habits. In reality, the user’s agency ultimately influences what content they engage with, illustrating the power of user preference in driving online polarization.
Causally estimating the effect of YouTube’s recommender system using counterfactual bots was published in Proceedings of the National Academy of the Sciences (PNAS)
AUTHORS
DELPHINE GARDINER
–
Communications Specialist