Originally published on the Empirica blog
Welcome to “Empirica Stories” — a series in which we highlight innovative research from the Empirica community, showcasing the possibilities of virtual lab experiments!
Today, we highlight the work of Levin Brinkmann and collaborators on their paper “Hybrid social learning in human-algorithm cultural transmission,” recently published in Philosophical Transactions of the Royal Society A.
Levin Brinkmann is a predoctoral fellow at the Center for Human and Machines at the Max Planck Institute for Human Development. In 2014, he received a Master in Physics from the University of Goettingen. He joined the center after working for several years as a data scientist in the fashion and advertising industry, where he developed inspirational algorithms for creative workers. Levin’s current interests are on the influence of algorithms on collective intelligence and on cultural evolution.
Tell us about your experiment!
There is anecdotal evidence that humans have reutilized solutions of an algorithm to their advantage. However, the scope and limits of such social learning between humans and AI are still unknown. To investigate this question, we ran an online study (N=177) with Empirica, in which participants solved a sequential decision-making task. We arranged participants in chains, such that participants’ solutions earlier in the chain could influence participants later in the chain. In some of the chains, an algorithm would take the place of a human participant, allowing us to observe the extent to which humans adopted the learnings from observing the solution generated by their algorithmic predecessor.
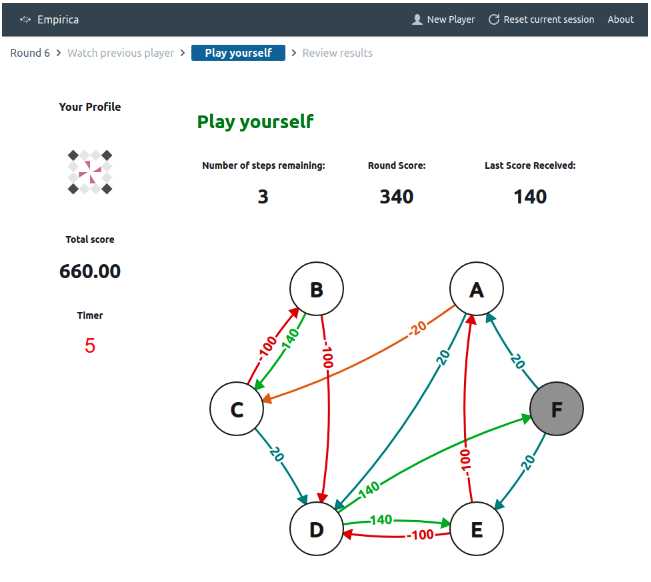
Screenshot of the experiment interface: Participants were tasked with traversing a network by choosing 8 successive nodes, and were incentivized to choose paths that maximized the cumulative score (each transition’s value is indicated by the arrow connecting the respective nodes). The player’s score updated in real-time as they moved from one node to the next.
What parts of Empirica’s functionality made implementing your experimental design particularly easy?
I love that Empirica is very opinionated, but at the same time, very versatile. The well-structured data model allows you to get started quickly. But at the same time, it is impressive how much customization is possible. We made a lot of use of the different event hooks provided by Empirica and they were all pretty much self-explanatory. For instance, we used a hook to pull a new chain and network structure from the database after each round, to display to the participant.
How much effort did it take to get your experiment up and running? Did you develop it or outsource?
Both — in our case, we developed the game interface ourselves, and had resources available to hire a developer to help us with the integration into Empirica. That allowed us to be 100% focused on the research question. Later on, we were able to make modifications to the code ourselves.
Are there any interesting workarounds you came up with when Empirica didn’t do quite exactly what you needed?
Our experiment relied on chains of sessions, which was a data model not natively supported by Empirica. However, it was relatively easy to add an additional data model for chains that suited our needs.
What value do you think virtual lab experiments can add to your field of research?
Generally, the scale of human interactions is increasing steadily, with interactions between humans and machines happening predominantly online. While we do work with data collected in “the wild”, I believe that controlled virtual lab experiments will remain the gold standard for research on human-AI interaction.
What’s next?
Since the writeup of this research, we have already started a new project using Empirica. In the new study, we run an interactive two player game and ask the question: “What mechanism do humans use to distinguish themselves from bots?”
—
Learn more about Empirica by visiting the Empirica website or our Group Dynamics project page.
AUTHORS
ABDULLAH ALMAATOUQ
—
Affiliated researcher
Massachusetts Institute of Technology