PIK professor Duncan Watts is out with an AI-enhanced tool to gauge the partisan lean and tone of some mainstream news outlets.
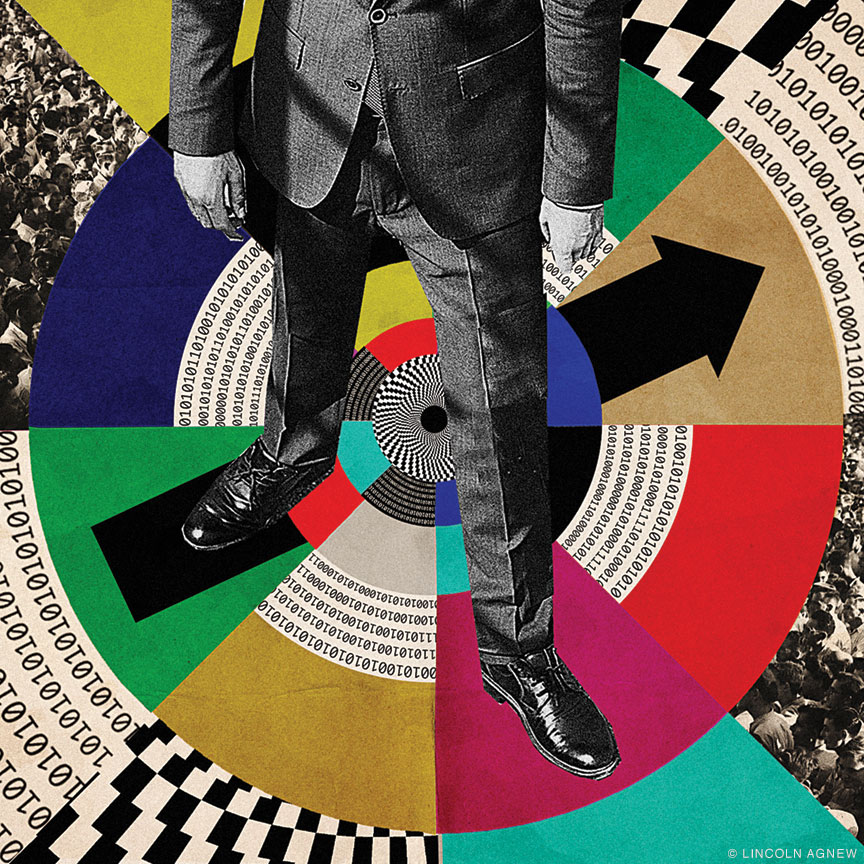
When Duncan Watts and his colleagues at Penn’s Computational Social Science Lab began work in January on a new tool that uses artificial intelligence to analyze news articles in the mainstream media, they aimed to release it before the presidential debate on June 27.
Originally published in
THE PENNSYLVANIA GAZETTE
August 26, 2024
“We built the whole thing from scratch in six months—which I’ve never experienced anything like in my academic career,” says Watts, the Stevens University Professor at the Annenberg School for Communication and the director of the Computational Social Science Lab (CSSLab). “It was a huge project, and a lot of people worked relentlessly to get it up.”
Knowing the debate between Joe Biden Hon’13 and Donald Trump W’68 could be critical in the presidential race, Watts hoped the Media Bias Detector would be up and running to help Americans understand the partisan lean of the outlets they get their news from leading into November’s election. “We just didn’t anticipate that the first debate would be such a dramatic turning point in the race,” he says.
Now, amidst a rollercoaster of seemingly endless political news highlighted by Kamala Harris replacing Biden at the top of the Democratic ticket, the website (mediabiasdetector.seas.upenn.edu) can “classify media content in somewhat close to real time,” says Watts, which he notes is “very unusual” in the world of academia where it often takes months to analyze data. Watts and his team do it by scraping articles from 10 online newspapers four times a day and pushing them through “this classification engine that we built that uses GPT” and can identify an article’s category, topic and subtopic; its tone (from very negative to neutral to very positive); and its political lean (from Democratic to Neutral to Republican). “Two years ago I would have said this can’t be done” says Watts, but today’s “transformer-based language models are wildly better than the last generation.”
A team of human research assistants (Penn undergraduates) monitor a random sample of labels generated by GPT to ensure that the model’s accuracy remains high. Watts also credits a project manager, data scientist, and “a bunch of really enterprising PhD students and postdocs” for building and maintaining the website itself, and the data journalism company Polygraph—“experts in visualizing data”—for creating the color-coded charts that help visitors make sense of editorial choices at the 10 featured outlets (including, for example, the percentage of articles each one devotes to politics—50 percent or more for all except the Wall Street Journal and USA Today.)
“Among the motivations for going through this exercise is that I think very often when we form impressions of the media or some particular publication, we’re really doing it based on a very small and highly selected sample of articles,” Watts says. “If you read all of them, you might reach a very different conclusion.”
Initially, Watts thought the Media Bias Detector would score or rank outlets based on their bias, but then realized “you can’t really measure bias directly,” he says. “We can’t compare each outlet to the truth, but we can compare them to each other. We can’t say how much they should have written about some issue, but we can say how much they wrote about that issue relative to some other issue. You, the reader, can reach your own conclusions about whether you think that’s a reasonable thing or not.” Watts’ team considered changing the name to something like the “Media Observer” to amplify the point that “we just count things.” But they kept the name because the goal to detect bias remains the same, and the site maintains a blog to analyze the data.
Watts has drawn some of his own conclusions from the data, albeit none that surprising. Most of the articles measured, including from the New York Times and Associated Press, have a negative tone. “People have known for a long time that the media has a negative bias, and that’s just really striking here,” Watts says. “But what’s even more striking is if you subset to politics, it gets very negative. So, you know, why does everybody think the country is in a bad way? Maybe it’s because that’s all the media talks about.” The charts also show the “enormous amount of attention paid to the horse race” between candidates, almost like it’s a sporting event, with far less reported about their stance on the issues. “It’s just breathless speculation and entertainment,” he says.
Watts has his own somewhat negative impressions of what the media chooses to report, recalling the “infuriating” decision by the New York Times to focus so much attention on the messy rollout of the Obamacare website in 2010 rather than “the tens of millions of people who were getting medical coverage that didn’t have it before, which to me was like the first, second, and third most important thing.” He also points to the buildup to the 2016 election when Hillary Clinton’s email controversy became the prevailing narrative of the campaign—which is one reason he would like to start gathering articles dating back to 2015 to measure media bias from a different time. He also hopes to add more news sources to the current list of 10 (the Associated Press, Breitbart News, CNN, Fox News, the Guardian, HuffPost, the New York Times, USA Today, the Wall Street Journal, and the Washington Post), as well as measuring more specific things like quotes that are used in articles.
While after the 2016 election “many other researchers immediately focused on social media, and in particular misinformation and fake news circulating on social media, our interests were drawn to the actual mainstream media,” Watts explains. “One reason for that is we have good evidence that it’s still what people overwhelmingly consume. The other reason is that you don’t need to lie to mislead people. … You can factually put accurate sentences together in a way that generates a very misleading impression.”
Although he admits that many readers might already know that most publications are in the business of “trying to get clicks,” he hopes visitors to the Media Bias Detector will internalize the data and cast a critical eye on “these publishers that adopt this pose of being unbiased and objective and comprehensive.”
“Everything that you learn about the world represents a choice that someone has made,” Watts says. “A journalist or an editor or a publisher has made the choice to focus on something and not something else. And so what you’re reading and what you’re learning is their opinion about what you should know.” —DZ
AUTHORS