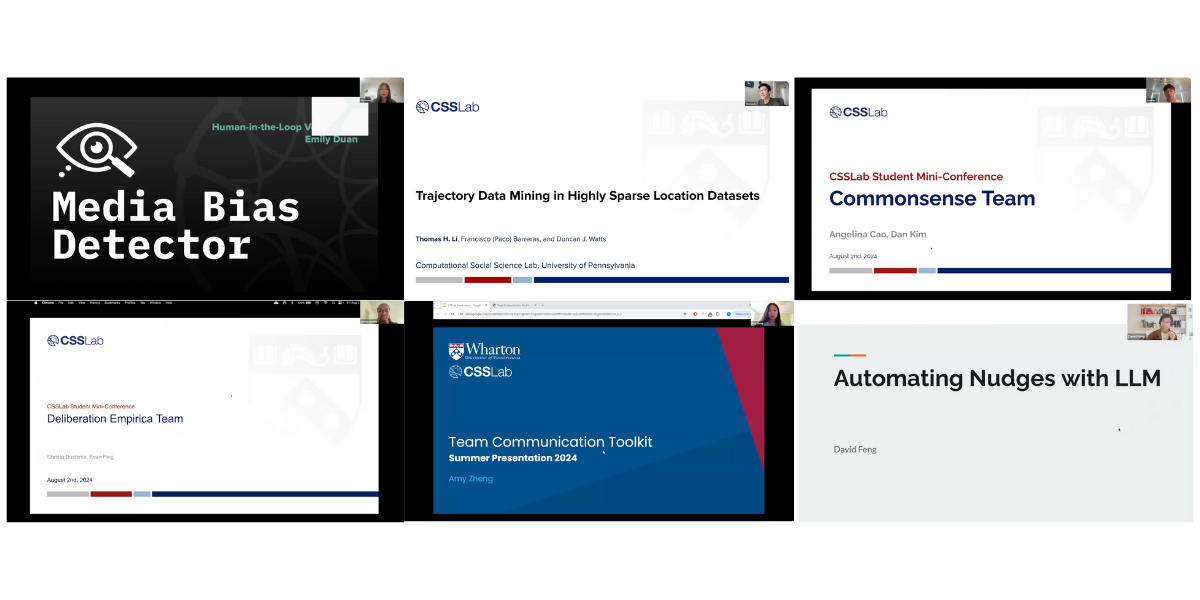
On August 2nd, ten undergraduate and Master’s students showcased their research at the third annual Student Research Mini-Conference, which featured presentations from all four major research groups at the Computational Social Science Lab (CSSLab) at Penn: PennMAP, COVID-Philadelphia/Human Mobility, Group Dynamics, and Common Sense. Here are the highlights from this conference:
PennMAP (Penn Media Accountability Project)
The Media Bias Detector is PennMAP’s latest tool designed to help people recognize bias in the mainstream media and to promote transparency and accountability in journalism in close to real-time. As the Media Bias Detector team was building this dashboard, some of the questions they wanted to address included: Why are topics like “Trump’s Legal Troubles” and “Biden’s Age” top stories in the news right now? and How do different publishers rank against one another when it comes to the tone/lean of “Trump’s Legal Troubles?” as they were interested in how media coverage varies between these publishers.
They find that media bias typically manifests in what events publishers choose to cover (selection) and how they present those stories (framing). Articles are retrieved from major publishers, and then GPT is used to classify each article by category/topic/subtopics, rate each article’s tone (negative, neutral, or positive) and lean (democrat, neutral, republican) and extract facts and quotes from each article.
However, recognizing that GPTs are imperfect, research assistant Emily Duan developed a human-in-the-loop platform to verify GPT’s article labels; through this system, research assistants evaluate the accuracy of these GPT-generated labels by manually verifying the labels GPT gives for selected articles. The research assistants also developed a master list of topics, which helps GPT classify articles into their respective categories; in addition, new topics and subtopics are continually added to this list to ensure that classification remains accurate.
COVID-Philadelphia/Human Mobility
The next research area is COVID-Phildelphia, which uses large-scale GPS data to model and understand human mobility movement. These GPS datasets provide detailed insights into human movement patterns, which can be used to identify epidemic hotspots, analyze behavior following large-scale disasters, and to study socioeconomic disparities in mobility data. While they offer great research potential, Thomas Li discusses a notable challenge that comes with these datasets: data sparsity. According to Thomas, data sparsity occurs when there are missing data points in these sets- this often leads to inaccurate conclusions about mobility data, such as misidentifying potential hotspots for transmission. Sparsity ultimately introduces bias, reducing the effectiveness of public health interventions as policymakers rely on accurate data to make informed decisions during epidemics.
To study sparsity, Thomas used synthetic data to create a miniature model for human mobility; it features zones, including parks, workplaces, retail places, and homes, with agents moving between these areas to stimulate realistic human movement. This model can also be adjusted for varying levels of data sparsity, such as how often data points are recorded. Having a controlled environment enables precise testing of these stop-detection algorithms and gives insights into how well these algorithms perform when available data is limited. Ongoing efforts are to refine this miniature simulation model and make it open-source, enabling other human mobility researchers to conduct more robust studies.
Common Sense
Common sense is often defined as “what all sensible people know,” but this definition is circular, as people believe they possess common sense but can’t specify which beliefs are considered common sense or whether others agree with their judgements. Dan Kim and Angie Cao worked on the Common Sense Project, where people can take an online survey to measure their own common sense by rating various statements. The statements come from diverse sources and will give researchers more insights into the nature and limits of common sense so that they can make comprehensive conclusions about collective common sense.
Dan worked to internationalize the Common Sense Project website by developing an application that enables localization for different target audiences across cultures and languages. This supports the survey in additional languages, including Arabic, Bengali, English, Spanish, French, Hindi, Portuguese, Russian, and Chinese, allowing the project to expand its database globally and gain a deeper knowledge on different populations.
Angie Cao focused more on the user experience and implemented a changed answers feature on the survey itself, which will allow users to change their survey responses and see how their answers compare to other participants in terms of what others think is common sense and how much other people agree with the given statements. Dan and Angie’s efforts will ensure that the CSSLab’s Common Sense project will reach a wider audience so that the research team can better understand common sense across diverse groups and participants can learn more about their shared beliefs with others.
Group Dynamics
There are three subgroups under the Group Dynamics research area: Group Deliberation, the Team Communication Toolkit, and Nudge Cartography.
Christa Dushime and Evan Ping talk about the Group Deliberation project; deliberation refers to when people discuss an issue to make decisions or resolve conflicts. The primary goal of Group Deliberation is to develop interventions to reduce affective polarization in group discussions, which would ultimately result in improved conversational outcomes, whether that is building mutual respect or solving collective problems. This is part of a larger online lab known as Empirica, where researchers can run their own virtual experiments to study these small-group interactions.
A Researcher Portal is underway so that social science researchers without a computer science background can still use the Group Deliberation services to develop and run their own polarization experiments. This portal would also allow for easy communication between different research groups, ensuring easy replication and comparison between experiments and community-building for those studying group dynamics.
The Team Communication Toolkit is a new tool in the form of a Python package that allows researchers to analyze text-based conversations among groups and teams by providing over a hundred research-backed conversational features. The toolkit can be used to better study communication; it is a valuable resource to answer communication questions in the areas of conflict, leadership, and negotiation, as the most predictive measure of a conversational outcome is about how team members communicate. Amy Zhang implemented a new feature into this toolkit called the “Named Entity Recognition,” which allows the toolkit to be able to identify and label objects, including names of people or locations mentioned in text conversations; having such features readily available in one place is beneficial to researchers so that they don’t have to build software from scratch every time they want to study specific conversational features.
Amy also worked on building the public-facing Team Communication Toolkit website, which introduces the project to users as well as provides the downloadable Python package and instructions on how to get the most out of the package. This approach is unique as studies on communication typically look at individual conversation features to predict conversational outcomes, but this package combines all of these features, which surprisingly revealed that in some cases interactions between multiple conversational features may lead to more productive conversational outcomes.
The last research area presented was Nudge Cartography, which involves mapping studies of the best nudges for specific situations. A nudge is defined as: “a person’s change in environment or context without restrictions to their choices or significantly altering their economic incentive,” or making “better” options more appealing but still giving them the freedom to choose. The CSSLab is especially interested in this relatively new field because it explores how subtle changes can influence one’s decision-making.
David Feng developed a website where users can upload messages (such as marketing messages), and an LLM automatically applies nudges to improve the effectiveness of the original message. David gave an example of inputting “We sell pizza to people,” but the LLM enhances the message by changing it to “We sell an authentic Italian experience,” which is more effective as it is projected to result in more sales than the original message. This automated approach not only shows the real-world uses of nudge cartography but also how nudges work in specific contexts, which will further inspire ongoing research into the field.
The CSSLab is thankful to the talented research assistants who presented their work to the lab members; their contributions have laid a foundation for ongoing research at the CSSLab. We would also like to acknowledge the other research assistants who did not present this year but are actively working on these projects alongside the PhD students and CSSLab researchers.
Some of these students were matched with the lab through the Penn Undergraduate Research Mentoring Program (PURM), a program by the Center for Undergraduate Research and Fellowships (CURF). Thank you to CURF (https://curf.upenn.edu/). More information about applying to PURM can be found here: https://curf.upenn.edu/content/penn-undergraduate-research-mentoring-program-purm
AUTHORS
DELPHINE GARDINER
–
Communications Specialist