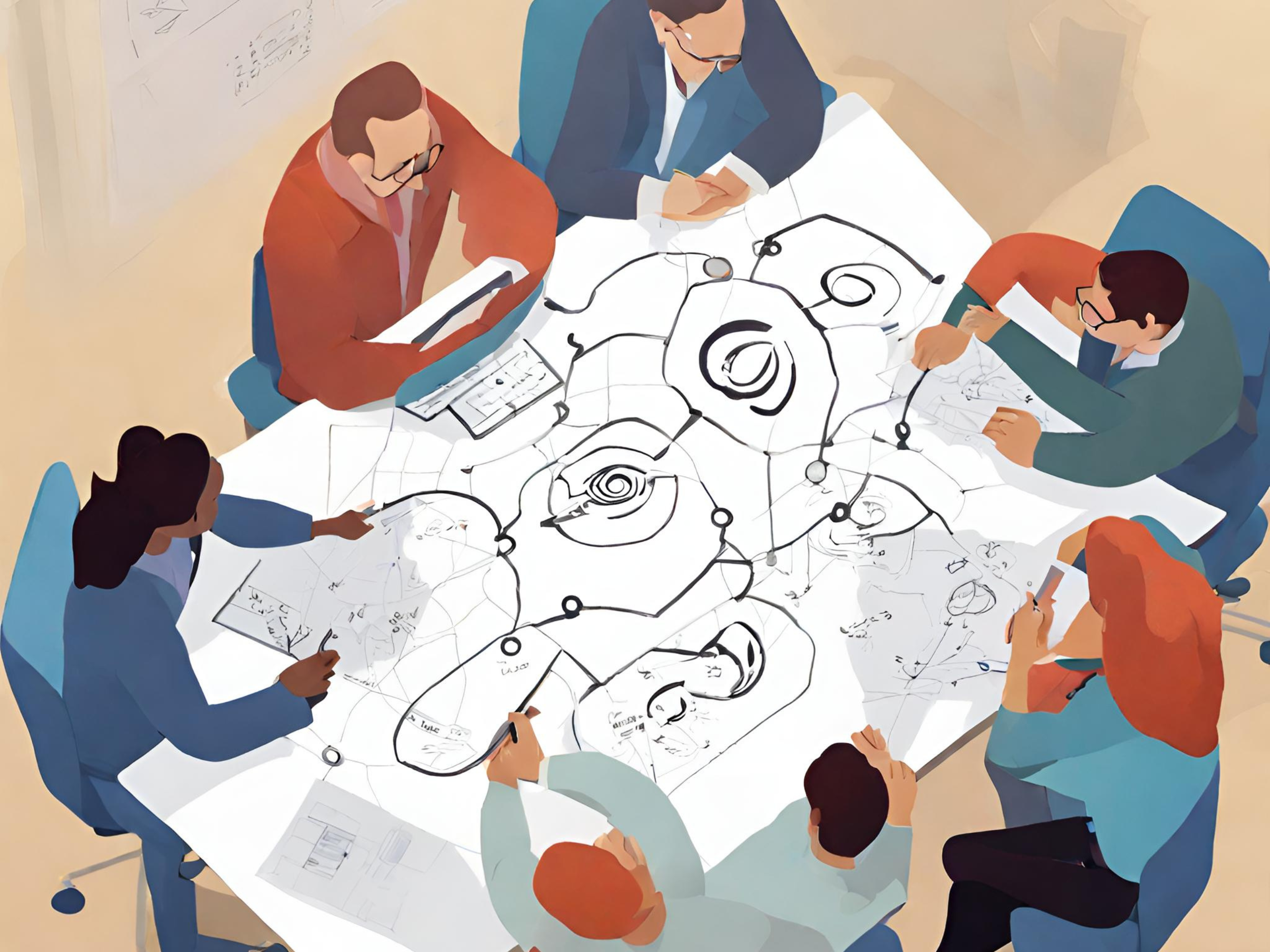
In the social and behavioral sciences, a theory provides a generalizable explanation that holds under a variety of specific conditions, and experiments are conducted to verify hypotheses which are derived from the theory. This process has become the dominant methodology under which scientific development occurs one experiment at a time, also known as the one-at-a-time approach.
However, coauthors Abdullah Almaatouq (Massachusetts Institute of Technology), Thomas L. Griffiths (Princeton University), Jordan W. Suchow (Stevens Institute of Technology), Mark E. Whiting (University of Pennsylvania), James Evans (University of Chicago, Sante Fe Institute), and CSSLab director Duncan J. Watts have proposed another approach which addresses the issues and inefficiencies associated with the integration of knowledge in the current model, called the integrative experimental design.
According to Almaatouq et al., the one-at-a-time approach is limited, as there is no standard to measure how similar or different experiments are to each other. Papers on group synergy demonstrate this problem, as many studies on group synergy have been carried out over several decades but with conflicting conclusions. In addition, the one-at-a-time approach is fixated on a single hypothesis, while the integrative experiment explores the possibility of several theories.
Moreover, theories derived from the one-at-a-time approach are rarely universally valid. For example, a paper can clearly state that a study pool includes students from a specific university but describes the results in a way that applies to the general public which misleads other researchers trying to compare studies. Therefore, this method fails to consider the diversity in behavioral patterns, and a solution would be to create an integrative design space for a whole range of possible experiments to yield data that is both useful and generalizable for real-world behavioral applications.
What would this integrative design space look like?
The construction of this design space involves research cartography, in which various research concepts and findings are mapped to reveal the relationship between them, like a “landscape.” This helps visually understand the association of different data types. To create a map of a theory you would identify all the relevant dimensions or variables that can contribute to the strength or occurrence of that topic. Each dimension would represent a coordinate (location) in the design space and these dimensions can be derived from literature and any known experiments on the theory.
As the number of identified dimensions increases, this also expands the size of the design space containing replicable experiments, resulting in an exponentially greater number of possible trials. Relatively small dimensions will allow scientists to exhaustively explore the space by researching all the possible trials. However, as the dimension becomes greater, the size of the design space increases dramatically to the point where it is no longer plausible to conduct all of them.
Fortunately, even in such a scenario, it is still possible to understand these experiments by using what is called active learning. The method iteratively selects the most informative design points to sample from the design space by coming up with optimal experimental design strategies. Then it uses what is called a surrogate model to predict all the outcomes of the design space without having to manually carry out each individual study. As a result, even the enormously large design space, which could not have been exhaustively explored, is now “understood” through predictions. This allows scientists to conduct research on the areas that the model predicts to have higher potential values.
Redefining Research
A possible concern with these large-scale integrative experiments is the higher cost to run them, but the benefit-cost ratio is greater as this approach eliminates the need to replicate experiments from scratch every time. Despite their intricacy, the integrative design allows researchers to facilitate mass collaboration with virtual labs by assigning different points in the design space to various groups to take on, encouraging participation from smaller labs and early-career researchers.
The integrative experimental design will not replace the one-at-a-time approach but instead fill in the gaps, as it emphasizes the importance of how theories are related to each other. Expanding the design space “map” also allows researchers to explore and discover new insights on research topics that could not have been achieved through human effort alone. This progress toward integration will give rise to cumulative, cohesive, and replicable research, strengthening the behavioral sciences research community.
The full paper can be found here.
AUTHORS
DELPHINE GARDINER
–
Communications Specialist