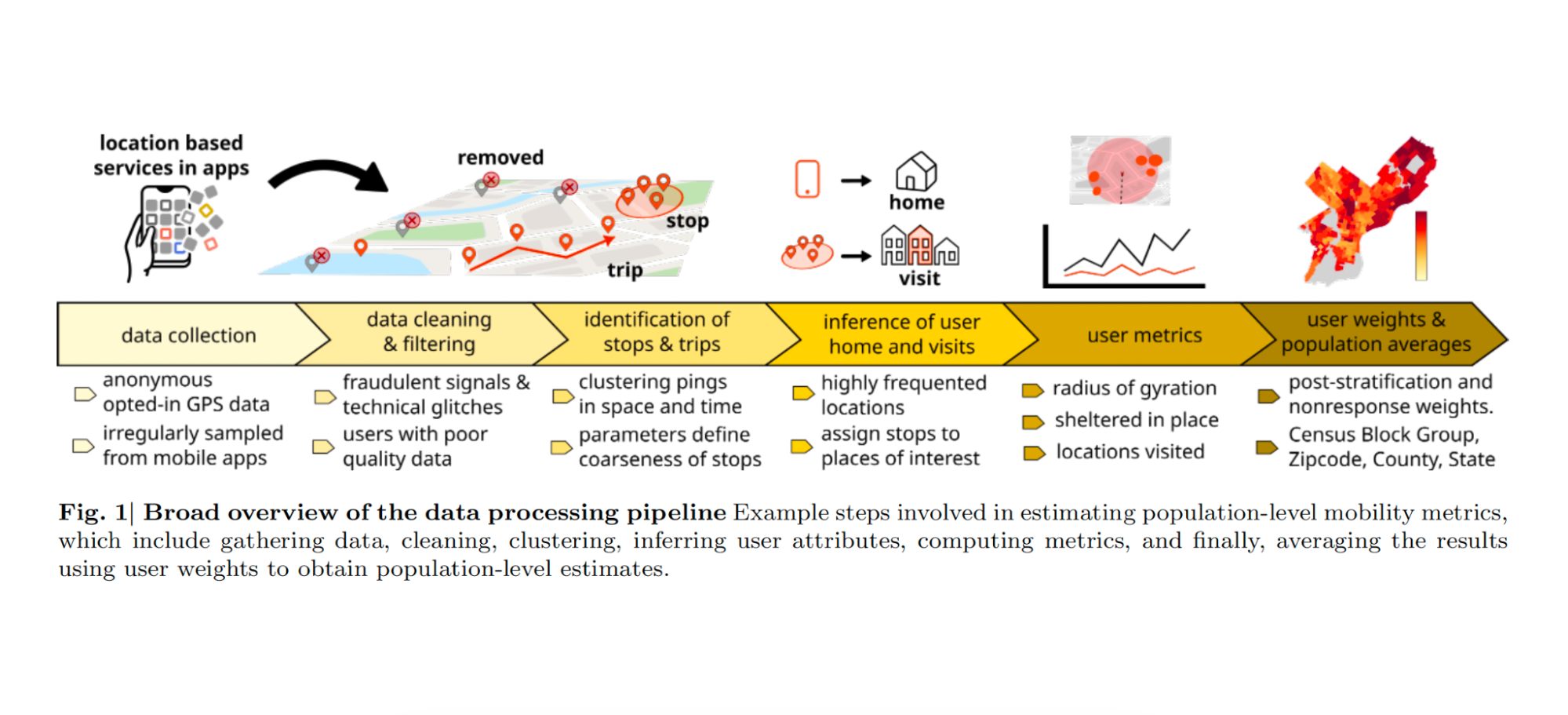
Epidemic modeling is a framework for evaluating the location and timing of disease transmission events, and is a part of the larger field of human mobility science. The COVID-19 pandemic put existing epidemic models to the test, with many institutions and corporations employing models that utilized smartphone location data to measure human-to-human interactions and better understand potential transmissions and social distancing.
Global Positioning System (GPS) data, aggregated into massive human mobility datasets—mined from thousands of smartphone apps in which users opt-in to location sharing—has an unprecedented precision that can revolutionize human mobility science, epidemiology in particular, and brings the promise of monitoring potential disease transmission events—in real time—during epidemics like COVID-19. Subsequently, researchers can determine if two people are at the same location at the same time and assess whether any two people in the same city have the chance to encounter one another and spread an infection.
Because this data is commercial, researchers interested in building epidemic models based on this data face limitations related to data privacy, quality and multiple methodological choices that might bias any findings. In their new paper, CSSLab Post-Doctoral Researcher Francisco (Paco) Barreras and CSSLab Director and Founder Duncan J. Watts discuss the nature and implications of these challenges. They determined that to improve the usefulness of this class of data, it is necessary to identify best practices from existing studies on human mobility, identify “sharp edges” in the data processing, and increase transparency and accessibility so that findings with important policy implications will be more robust.
Concerns About Privacy
Because human mobility patterns are predictable (due to humans’ “circadian cycles”), removing identifying information from GPS data is not sufficient to protect user privacy; even if individual names are removed from data it is still possible to identify most people based on their four most visited locations.
This concern results in many companies privatizing their data by heavily restricting access to individual-level data. In Barreras’s and Watts’s experience, accessing this data “was a costly and lengthy process, requiring guidance from a legal team as well as technical expertise to process the data itself, so acquiring a single dataset would take several months at a time.” Faced with such high barriers to entry, it is unsurprising that most researchers prefer using pre-computed metrics by data providers that have been sufficiently aggregated to protect privacy.
Paco Barreras, Ph.D.
Despite these very real privacy concerns, Barreras notes that “There are viable solutions. Companies like Spectus are leading the effort in facilitating privacy-centered analyses with in-house solutions—secure platforms where the data never leaves the company’s servers; instead allowing researchers to input their own code and only get the aggregated results. We need community-led solutions of this type.” This method reduces the exposure to bad actors and, in combination with privacy-aware methodologies, can prevent the re-identification of individuals.
Processing Human Mobility Data
Processing the “raw” data, long streams of deidentified “pings” at the level of users (datasets that are often several Terabytes in size), requires a complex pipeline before it can be turned into meaningful mobility metrics (such as movement patterns or how many times a person visits a location). This is useful to researchers who can then make inferences about patterns at large, make predictions, or formulate interventions.
But Barreras and Watts note that there is a wide array of ways to process human mobility data which can result in inconsistent conclusions even when working with the same dataset. The authors reference Jorge Luis Borges’s concept of a “garden of forking paths,” where “any one set of choices appears reasonable when viewed in isolation, but where very different conclusions may be obtained by making equally reasonable but different choices.” They find that in various stages of processing, researchers must watch out for the following potential sources of error–sparsity, bias, and lack of algorithm robustness– that can inadvertently skew the results.
Data sparsity occurs when there are long gaps without data in the GPS trajectories of some users, at times excluding entire subpopulations from an analysis. To demonstrate this problem, suppose you want to know how people explore cities and you yourself might be interested in the number of unique locations you’ve visited. But if you only observe 10% of your activity, how would you estimate the total number of locations you’ve actually been to? “Simply interpolating by multiplying by 10 to get the total number of unique locations you visited seems wrong, but any other solution requires critical assumptions” notes Barreras.
This data missingness problem is also an equity issue since in the majority of the developing world, there is less than perfect cellular and internet coverage, and having a smartphone can still be considered a luxury. Thus, the benefits and developments in epidemiology that leverage this class of data would disproportionately benefit the countries and areas with data of better quality, neglecting the places where these benefits are needed the most.
Another source of error is bias, emerging naturally from how the data is collected. If restaurants are overrepresented in the dataset of a company serving ads for the food industry, can people really trust the conclusion that restaurants were a main driver of infections during COVID-19? There is literature pointing at restaurants as the more dangerous businesses to reopen in terms of resulting infections, so, if a policymaker read this work, they could proceed to shut down restaurants for longer as a result. Very few people need convincing that epidemic-related policies are high-stakes, thus, recognizing bias is imperative to ensure that decisions made are evidence-based.
Robustness, the last source of error, refers to how simple choices regarding the parameters of algorithms can introduce errors in the resulting metrics— perhaps inflating the estimates of time spent at home, or ignoring the visits to small locations. Barreras’s experience was that, upon receiving a huge amount of initial data, he realized that even long before any analyses could be conducted, simple choices, like how to filter out incomplete users or “clean” the data, carried computational costs and could be done in a multitude of ways. “When I looked at the literature, I didn’t find a lot of guidance, as most methodologies were different or undisclosed,” Barreras says.
When navigating the avalanche of COVID-19 studies, the authors found that studies with similar goals would tackle the challenges of the data with creative and defensible solutions, “unfortunately very different from paper to paper,” says Barreras. Some papers do not fully disclose their pre-processing methods, reducing transparency and making replicability nearly impossible. An example in their paper references Safegraph, a location data company, which published an estimate of the number of people staying at home completely during the COVID-19 lockdown in the United States. This data seemed to confirm that stay-at-home mandates were highly effective at increasing distancing, however, a later release of 2019 data showed an implausibly high number of households (upwards of 60% in some neighborhoods in Philadelphia) were staying at home completely before the pandemic, and that the values in January and February of 2020 were anomalously low.
These puzzling anomalies, possibly related to methodology changes that only were applied to newer releases of the data, raised concerns about the validity of a myriad of applications that employed this time series for monitoring and predictions. “These are the types of errors that can be prevented if the methodology is accessible to independent researchers that can scrutinize it and replicate it,” say the authors, who envision a community-maintained repository of mobility metrics that are transparent and can be trusted.
Improving GPS Human Mobility Data
Following their findings, Barreras and Watts discuss potential next steps. They recommend building repositories where relevant documentation and datasets can be found in a central location to improve the replicability and accessibility of data to researchers. Then the standardization of methodologies will establish guidelines for processing data and promote transparency. Additionally, having a system in place to validate human mobility data and gain access to data from marginalized regions would ensure that the results are unbiased, addressing inequities in the field.
The authors hope that these suggestions can encourage community-level dialogue on obtaining the most robust and reliable results for developing epidemic models, as responses to the COVID-19 pandemic reflect flaws in these models as well as our vulnerabilities to infectious disease. They emphasize that future research and efforts to improve data quality should not just be for the sake of academic pursuits but also to improve policymakers’ responses to public health crises.
The exciting potential and daunting challenge of using GPS human-mobility data for epidemic modeling was published in Nature Computational Science
In related news, Barerras and Watts have recently received a grant “Democratizing Access to Large-Scale GPS Location Data and Processing Algorithms for Epidemic Modeling” from the Human Networks and Data Science program of the National Science Foundation (NSF). This grant will enable them to implement some of the initiatives proposed in their paper; in particular, building a platform to facilitate researcher access to multiple datasets and algorithms.
AUTHORS
Delphine Gardiner
—
Senior Communications Specialist