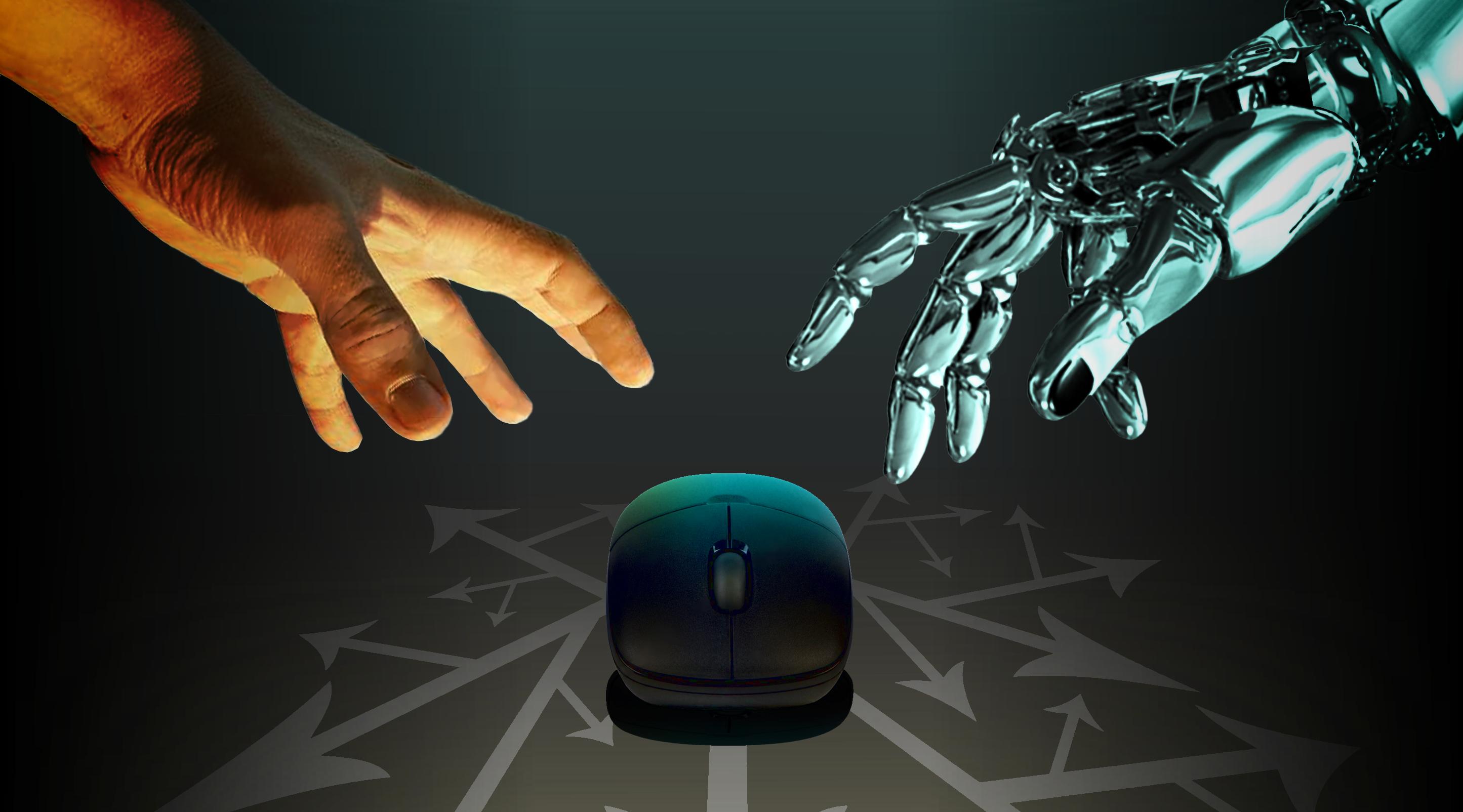
The past two election cycles have drawn new attention to the internet’s impact on democracy. With political polarization on the rise and trust in traditional sources of authority on the decline, concerns have mounted over the distribution of false, hyperpartisan, and conspiratorial content on social media.
However, relatively little research has focused on YouTube’s role in recent polarization. Most studies on this aspect of social media have focused on Facebook and Twitter, despite the roughly 23 million Americans relying on YouTube as a source of news. Not only is this audience comparable to the corresponding Twitter population, but it is growing in both size and engagement—facts which, combined with anecdotes of YouTube steering users towards radical content, have raised new concerns over the YouTube recommendation algorithm’s potential radical bias.
In their recent paper, Homa Hosseinmardi et al. examine these claims using a data-driven approach. The results indicate that YouTube’s recommendation algorithm may not be the radicalization pipeline that earlier literature suggests. Rather, their work highlights the need to understand the web more holistically, with YouTube being just one part of a larger ecosystem for distributing, discovering, and consuming political content.
Examining YouTube in context
The authors’ method relies on large-scale data to get a sense of systemic trends. Using a nationally representative web panel, they analyzed the individual-level browsing behavior of more than 300,000 YouTube users, yielding nearly 10 million unique video IDs.
Categorizing this substantial panel data provided a broad view of how users interact with different kinds of political content. The authors labeled nearly a thousand YouTube channels—along with their corresponding videos—according to their position along a political spectrum: far-left, left, center, right, far-right, and broadly “anti-woke.” Finally, grouping users’ viewing behavior into sessions—or sets of near-consecutive YouTube pageviews—allowed them to examine how interactions with political content on YouTube overlapped with similar interactions off of the platform.
Systemic bias or user preference?
A key finding is the prevalence of clusters, or communities, of viewers with relatively distinct and homogenous consumption preferences. These communities align closely with the six content categories used in the research. While the dominant trend was for viewers to remain in their communities from month to month—suggesting community “stickiness”—Hosseinmardi et al. observed trends in migration between communities as well.
How can we tell whether the YouTube platform itself drives these migration patterns? To clarify the potential causes of radicalization, Hosseinmardi et al. tested whether the recommendation algorithm was to blame in three ways.
First, they examined how a user’s consumption of political content on YouTube compared to their consumption of similar content off of the platform. If the algorithm does drive radicalization through its recommendations, they would expect to see more interaction with radical political content on the platform compared to off of it. Instead, the authors found a strong correlation between on- and off-platform tastes. This indicates that, while the algorithm cannot be completely ruled out as a factor, radical content consumption on YouTube is based largely on user preferences.
Their second strategy was to analyze the pathways that viewers used to find radical content on YouTube. If the recommender does determine viewing patterns, the authors would expect to find that arrivals at radical content are dominated by the video views immediately preceding them. However, they observed that other sources played key roles. For instance, while 36% of far-right videos were preceded by another video, nearly 55% of referrals came from external URLs, the YouTube homepage, or direct searches. While this, too, does not entirely rule out the recommendation system, it emphasizes the consistency of user preferences across platforms.
Finally, the authors checked whether radical content was more likely to be consumed later in a user’s viewing session, after viewers had been exposed to more recommended content. A biased recommendation algorithm would lead to an increased frequency of radical content towards the end of a session, but they observed the opposite—all six content categories showed decreasing frequency as sessions progressed, suggesting that longer viewing sessions are increasingly steered towards non-news content. This undercuts claims of YouTube driving radicalization through its recommendation system.
Future work
Rather than indicate algorithmic bias, these patterns of behavior showed that YouTube is just one of many “libraries” that supply radical political content. Individual-level radicalization was still observed in the study. However, Hosseinmardi et al. found little evidence that the platform’s recommendation system pushes viewers towards radical videos, instead suggesting that user preferences are consistent with their consumption outside of YouTube. In addition to finding no evidence of systemic bias, the authors note that examining radical content on its own is limiting, and a broader investigation of media consumption—including on mobile platforms—is needed to better understand online radicalization.
CSS Lab is excited to see other research on the platform’s impact on politics and public trust. Since Hosseinmardi et al. examine trends in consumption across the political spectrum, building off of this research may involve deeper analyses of the far-left, far-right, and anti-woke communities in particular. Their work sets the stage for a closer, data-driven look at video-based news content more broadly, allowing for a better understanding of how political attitudes are shaped.
For more details, read the full paper published in PNAS here.
Anonymized CSV data used in this research can be accessed through the Open Science Framework (OSF) here.
AUTHORS
EMMA ARSEKIN
—
Communications Specialist